A Siamese Network-Based Contrastive Learning Image Quality Assessment Method
An image quality assessment, twin network technology, applied in biological neural network model, image analysis, image data processing and other directions, can solve the problem of inaccurate labeling, algorithm performance depends on the performance of full reference image quality evaluation, and the quality difference of different parts of the image. and other problems, to achieve the effect of strong representation ability and improvement of quality evaluation performance
- Summary
- Abstract
- Description
- Claims
- Application Information
AI Technical Summary
Problems solved by technology
Method used
Image
Examples
Embodiment Construction
[0032] The technical solution of the present invention will be specifically described below in conjunction with the accompanying drawings.
[0033] The present invention provides a comparison learning image quality assessment method based on Siamese network, such as figure 1 shown, including the following steps:
[0034] Step S1: Perform local contrast normalization processing on the image to be trained, then divide it into image blocks, and generate image pairs.
[0035] Step S11: first perform local contrast normalization on all distorted images, and calculate the normalized value for a given intensity image I(i,j) The formula is as follows:
[0036]
[0037]
[0038]
[0039] Among them, C is a constant used to prevent the denominator from being zero; K and L are the normalized window size, ω k,l is a 2D circular symmetric Gaussian weighting function;
[0040] Step S12: Divide all local contrast normalized images into several image blocks of size h×w, sort all...
PUM
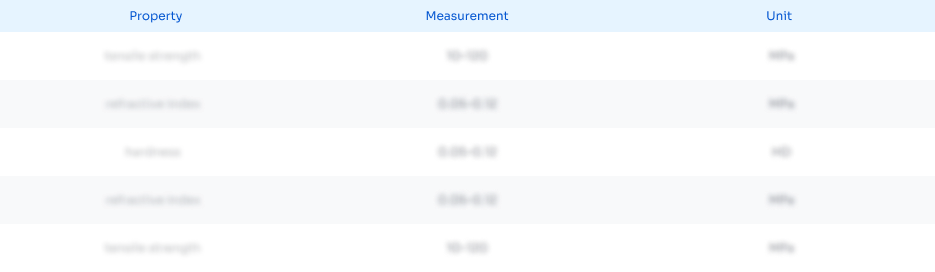
Abstract
Description
Claims
Application Information
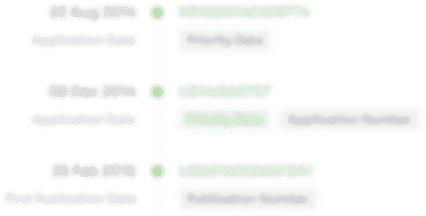
- R&D Engineer
- R&D Manager
- IP Professional
- Industry Leading Data Capabilities
- Powerful AI technology
- Patent DNA Extraction
Browse by: Latest US Patents, China's latest patents, Technical Efficacy Thesaurus, Application Domain, Technology Topic, Popular Technical Reports.
© 2024 PatSnap. All rights reserved.Legal|Privacy policy|Modern Slavery Act Transparency Statement|Sitemap|About US| Contact US: help@patsnap.com