Rolling bearing fault diagnosis method based on parallel feature learning and multiple classifiers
A rolling bearing and multi-classifier technology, applied in the fields of instruments, character and pattern recognition, computer parts, etc., can solve the problems of not being able to filter out deep features, affecting the accuracy of model classification and diagnosis, and evaluating deep features.
- Summary
- Abstract
- Description
- Claims
- Application Information
AI Technical Summary
Problems solved by technology
Method used
Image
Examples
Embodiment Construction
[0041] The following describes the present invention in further detail with reference to the drawings and specific embodiments:
[0042] Reference figure 1 , The present invention includes the following steps:
[0043] Step 1) Obtain training sample set and test sample set
[0044] A total of 12 fault types and 3,600 vibration time-domain signals of rolling bearings are collected through the data collection system as a data set, 2400 of which are used as the training set, and the remaining 1,200 data are used as the test set, as follows:
[0045] The vibration time domain signals used in this embodiment all come from the bearing vibration time domain signals collected by the bearing accelerated life test bench PRONOSTIA. The platform consists of three parts: drive module, load module and data acquisition module. The main function of the test device is to provide signals of different types of faults. The main components of the test device include a drive motor, a torque sensor and a ...
PUM
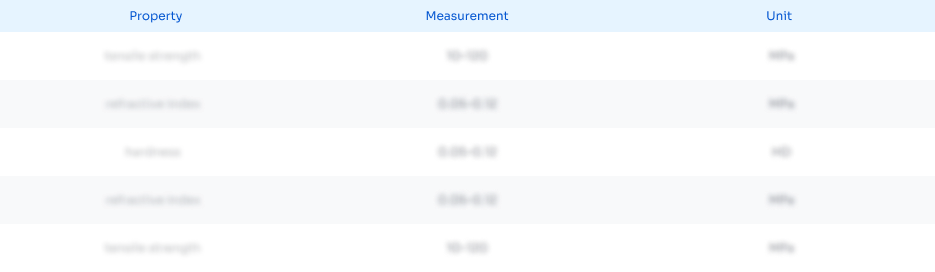
Abstract
Description
Claims
Application Information
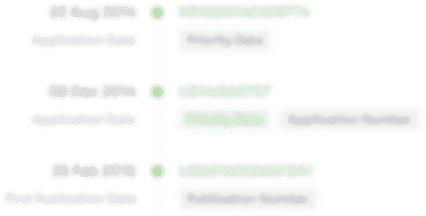
- R&D
- Intellectual Property
- Life Sciences
- Materials
- Tech Scout
- Unparalleled Data Quality
- Higher Quality Content
- 60% Fewer Hallucinations
Browse by: Latest US Patents, China's latest patents, Technical Efficacy Thesaurus, Application Domain, Technology Topic, Popular Technical Reports.
© 2025 PatSnap. All rights reserved.Legal|Privacy policy|Modern Slavery Act Transparency Statement|Sitemap|About US| Contact US: help@patsnap.com