A Steady State Visual Evoked Potential Signal Classification Method Based on Convolutional Neural Network
A convolutional neural network and steady-state visual induction technology, applied in biological neural network models, neural architecture, medical science, etc., can solve the limitations of SSVEP-BCI engineering applications, individual differences are not considered, and the recognition accuracy is low problem, to achieve the effect of improving application performance, adapting to individual differences, and high information transmission rate
- Summary
- Abstract
- Description
- Claims
- Application Information
AI Technical Summary
Problems solved by technology
Method used
Image
Examples
Embodiment Construction
[0031] The present invention will be further described in detail below in conjunction with the accompanying drawings and embodiments.
[0032] Such as figure 1 As shown, a steady-state visual evoked potential signal classification method based on convolutional neural network, including the following steps:
[0033] Step 1, such as figure 2 As shown in (a), when four stimulation targets moving at different cycle frequencies are presented on the monitor at the same time, the frequencies of the four stimulation targets are left 6 Hz, right 7 Hz, upper 8 Hz, and lower 9 Hz, and the design and presentation of the stimulation targets are uniform Implemented by the Psychtoolbox toolbox based on MATLAB;
[0034] Step 2, the user chooses to focus on a specific target, and at the same time uses the EEG signal acquisition instrument to collect the SSVEP signal of the user at this time. According to the international standard 10 / 20 system method, the SSVEP signal collects visual brain ...
PUM
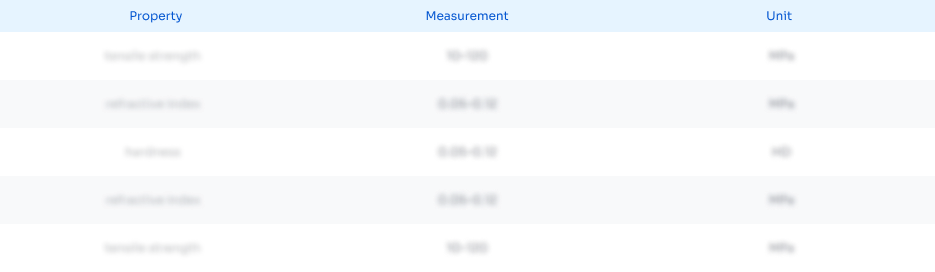
Abstract
Description
Claims
Application Information
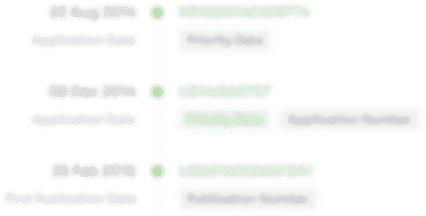
- R&D Engineer
- R&D Manager
- IP Professional
- Industry Leading Data Capabilities
- Powerful AI technology
- Patent DNA Extraction
Browse by: Latest US Patents, China's latest patents, Technical Efficacy Thesaurus, Application Domain, Technology Topic, Popular Technical Reports.
© 2024 PatSnap. All rights reserved.Legal|Privacy policy|Modern Slavery Act Transparency Statement|Sitemap|About US| Contact US: help@patsnap.com