A two-stream network action recognition method based on spatio-temporal saliency action attention
An attention and sexual behavior technology, applied in character and pattern recognition, biological neural network models, instruments, etc., can solve problems such as difficulty in ensuring the activity and availability of effective information, improve behavior recognition efficiency, reduce storage pressure, and implement Efficient effect
- Summary
- Abstract
- Description
- Claims
- Application Information
AI Technical Summary
Problems solved by technology
Method used
Image
Examples
Embodiment Construction
[0031] figure 2 It represents the algorithm model diagram of the present invention. The algorithm takes RGB keyframes and optical flow keyframes as input, and the model includes six key parts: salient behavior detection network, attention network, spatial network, temporal network, classification and fusion. The spatial network uses a bidirectional LSTM architecture, while the temporal network uses a C3D architecture. Finally, the weighted average fusion method is used to fuse the two networks, and the default fusion weights of the two streams are 0.5 respectively.
[0032] In order to better illustrate the present invention, the following takes the public behavior data set UCF101 as an example.
[0033] The data processing method of the key frame mechanism in step 3 in the above technical solution is:
[0034] Traditional behavior recognition methods usually take frames at random or segmented frames. The present invention introduces a video summarization method to extract ...
PUM
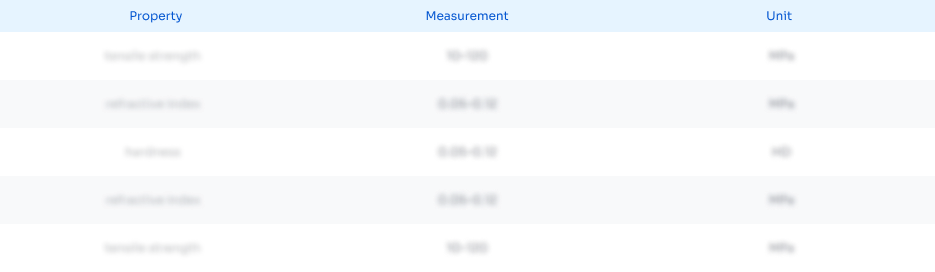
Abstract
Description
Claims
Application Information
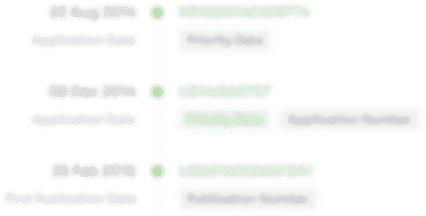
- R&D Engineer
- R&D Manager
- IP Professional
- Industry Leading Data Capabilities
- Powerful AI technology
- Patent DNA Extraction
Browse by: Latest US Patents, China's latest patents, Technical Efficacy Thesaurus, Application Domain, Technology Topic, Popular Technical Reports.
© 2024 PatSnap. All rights reserved.Legal|Privacy policy|Modern Slavery Act Transparency Statement|Sitemap|About US| Contact US: help@patsnap.com