Named entity identification method based on adversarial training
A technology for named entity recognition and training samples, which is applied in special data processing applications, instrumentation, semantic tool creation, etc., can solve the problems of model performance loss, limited number of texts, large data scale, etc., and achieve enrichment of training samples, The effect of increasing generalization ability and robustness
- Summary
- Abstract
- Description
- Claims
- Application Information
AI Technical Summary
Problems solved by technology
Method used
Image
Examples
Embodiment Construction
[0026] The present invention will be described in detail below with reference to the accompanying drawings and examples.
[0027] The present invention provides a named entity recognition method based on adversarial training, such as Figure 4 As shown, the specific process is as follows:
[0028] Step 1. The present invention introduces the RoBERTa model in the judicial field. First, it performs corresponding word segmentation for each judicial field text, and inputs it into RoBERTa in the form of characters, and assigns different words to different words through the self-attention mechanism (self-attention). The weight of , that is, assuming that the input matrix is X, the largest word embedding vector is 512, through different weight matrices W q , W k , W v , and finally obtain the self-attention matrix Z through softmax, and obtain multiple representation subspaces of the attention layer through the multi-head mechanism, and finally stitch different matrices Z, and e...
PUM
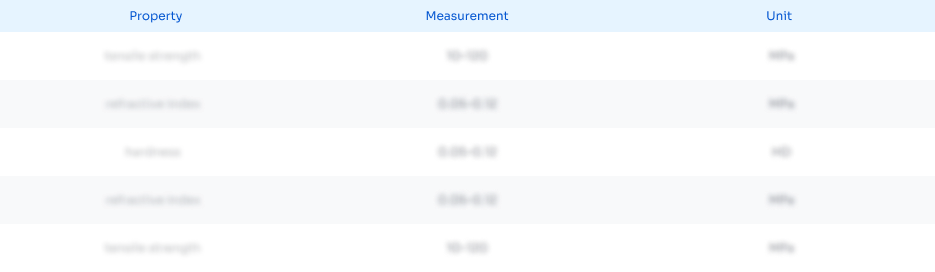
Abstract
Description
Claims
Application Information
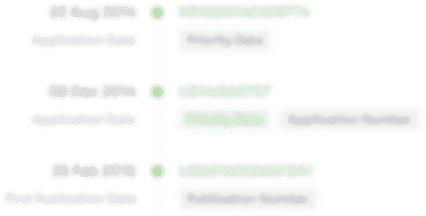
- R&D Engineer
- R&D Manager
- IP Professional
- Industry Leading Data Capabilities
- Powerful AI technology
- Patent DNA Extraction
Browse by: Latest US Patents, China's latest patents, Technical Efficacy Thesaurus, Application Domain, Technology Topic, Popular Technical Reports.
© 2024 PatSnap. All rights reserved.Legal|Privacy policy|Modern Slavery Act Transparency Statement|Sitemap|About US| Contact US: help@patsnap.com