Adaptive convolution residual error correction single image rain removal method
A residual error correction and single image technology, applied in the field of image processing, can solve problems such as increasing network expression, rain line residue, background loss, etc.
- Summary
- Abstract
- Description
- Claims
- Application Information
AI Technical Summary
Problems solved by technology
Method used
Image
Examples
Embodiment Construction
[0066] The specific embodiment of the present invention will be further described below in conjunction with accompanying drawing:
[0067] This embodiment mainly provides a method for removing rain from a single image with adaptive convolution residual correction, including the following steps:
[0068] S1) Data collection, the data set is divided into two parts: training data set and test data set.
[0069] In the training phase, it is necessary to provide the convolutional network with a rainy map and its corresponding non-rainy map. In the actual collection process, it is very difficult to directly obtain pictures of the same scene without rain and with rain, because even if the position of the camera can be kept completely unchanged, the surrounding environmental conditions such as brightness when shooting will also be different. The existing deep learning-based rain removal algorithms all use synthetic rain maps to train the network. The rain-free image is added with ra...
PUM
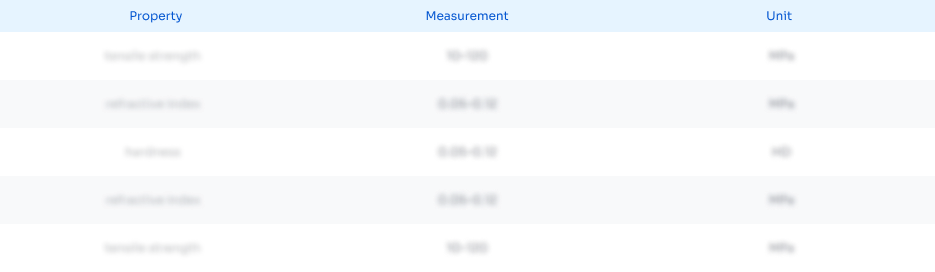
Abstract
Description
Claims
Application Information
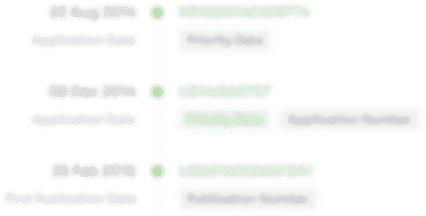
- R&D Engineer
- R&D Manager
- IP Professional
- Industry Leading Data Capabilities
- Powerful AI technology
- Patent DNA Extraction
Browse by: Latest US Patents, China's latest patents, Technical Efficacy Thesaurus, Application Domain, Technology Topic, Popular Technical Reports.
© 2024 PatSnap. All rights reserved.Legal|Privacy policy|Modern Slavery Act Transparency Statement|Sitemap|About US| Contact US: help@patsnap.com