Porous medium image reconstruction method based on generative network
A technique for porous media and image reconstruction, applied in the field of image processing, can solve problems such as difficulties, missing, incomplete 3D reconstruction and analysis of training images, and achieve fast and accurate reconstruction
- Summary
- Abstract
- Description
- Claims
- Application Information
AI Technical Summary
Problems solved by technology
Method used
Image
Examples
Embodiment
[0037] In order to make the reconstruction method described in the present invention easier to understand and closer to the real application, the following is a detailed overall description of each step of the reconstruction method based on deep learning. The specific operation steps are as follows:
[0038] (1) For different reconstructed images, make a data set for deep neural network training. image 3 Several samples from the dataset of rubber / silica materials are given (where white is silica and black is rubber). The data set has a total of 800 image pairs, and each image pair consists of two 128×128 images to be reconstructed and the target image.
[0039] (2) The calculation of the mode-based constraint function is as follows: Figure 4 shown. In the experiment, in order to balance the accuracy and speed of reconstruction, a template size of 3×3 is used. That is to say, there are at most 2 types of patterns 3×3 = 512 patterns. Count the frequency of these patterns ...
PUM
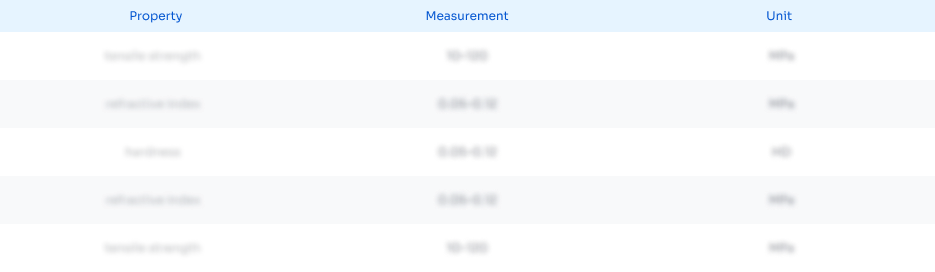
Abstract
Description
Claims
Application Information
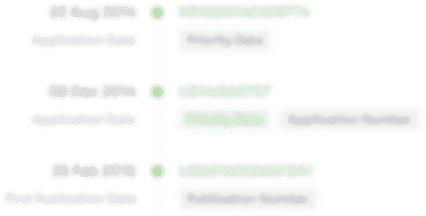
- R&D Engineer
- R&D Manager
- IP Professional
- Industry Leading Data Capabilities
- Powerful AI technology
- Patent DNA Extraction
Browse by: Latest US Patents, China's latest patents, Technical Efficacy Thesaurus, Application Domain, Technology Topic.
© 2024 PatSnap. All rights reserved.Legal|Privacy policy|Modern Slavery Act Transparency Statement|Sitemap