Multi-source Remote Sensing Image Classification Method Based on Robust Deep Semantic Segmentation Network
A technology of semantic segmentation and remote sensing image, applied in the field of robust learning of remote sensing semantic segmentation, which can solve the problems of adverse effects of model learning and low accuracy of classification results, and achieve the effect of reducing sample labeling work and eliminating adverse effects.
- Summary
- Abstract
- Description
- Claims
- Application Information
AI Technical Summary
Problems solved by technology
Method used
Image
Examples
Embodiment Construction
[0038] In order to facilitate those of ordinary skill in the art to understand and implement the present invention, the present invention will be described in further detail below in conjunction with the accompanying drawings and embodiments. It should be understood that the implementation examples described here are only used to illustrate and explain the present invention, and are not intended to limit this invention.
[0039] please see figure 1 , the present invention provides a multi-source remote sensing image classification method based on a robust deep semantic segmentation network, which consists of a fault-tolerant loss function and an adaptive category weight. Include the following steps:
[0040] Step 1: Deep semantic segmentation network training and object classification. The remote sensing image data set is used as the input data of the deep semantic segmentation network for training. The loss function in the deep semantic segmentation network is the RSRLF rob...
PUM
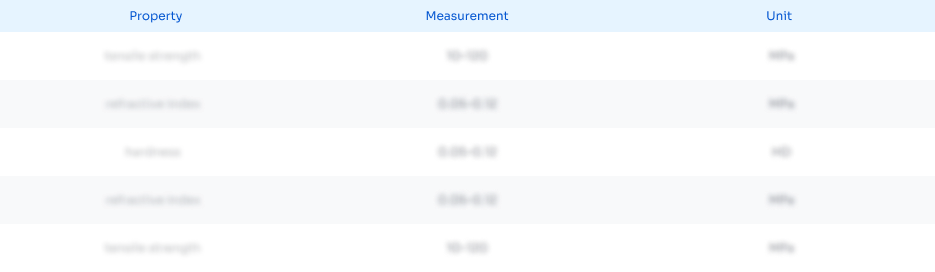
Abstract
Description
Claims
Application Information
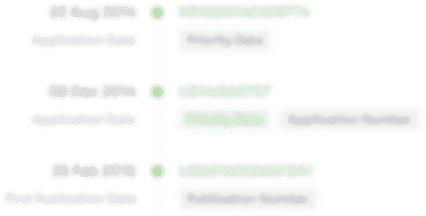
- R&D Engineer
- R&D Manager
- IP Professional
- Industry Leading Data Capabilities
- Powerful AI technology
- Patent DNA Extraction
Browse by: Latest US Patents, China's latest patents, Technical Efficacy Thesaurus, Application Domain, Technology Topic, Popular Technical Reports.
© 2024 PatSnap. All rights reserved.Legal|Privacy policy|Modern Slavery Act Transparency Statement|Sitemap|About US| Contact US: help@patsnap.com