Hyperspectral image classification method based on deep learning space-spectrum joint network
A hyperspectral image and joint network technology, applied in the field of hyperspectral image classification, can solve the problems of incomplete extraction of spectral information, destruction of context continuous information, and inability to extract feature information, etc., to improve the final classification accuracy, distinguish features meticulously, The effect of enriching discriminative features
- Summary
- Abstract
- Description
- Claims
- Application Information
AI Technical Summary
Problems solved by technology
Method used
Image
Examples
Embodiment Construction
[0025] Below in conjunction with accompanying drawing and specific embodiment, further illustrate the present invention, should be understood that these embodiments are only for illustrating the present invention and are not intended to limit the scope of the present invention, after having read the present invention, those skilled in the art will understand various aspects of the present invention Modifications in equivalent forms all fall within the scope defined by the appended claims of this application.
[0026] Such as figure 1 As shown, the present invention discloses a hyperspectral image classification method based on deep learning space-spectrum joint network. First, the original hyperspectral image is divided into data, and then a small amount of labeled data is used to train the space-spectrum joint network based on deep learning, and finally the trained network parameters are combined for classification. In the deep learning space-spectrum joint network, the inpu...
PUM
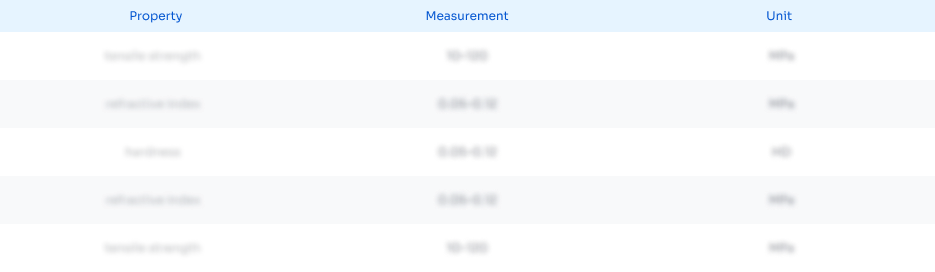
Abstract
Description
Claims
Application Information
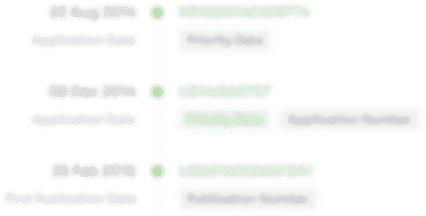
- R&D
- Intellectual Property
- Life Sciences
- Materials
- Tech Scout
- Unparalleled Data Quality
- Higher Quality Content
- 60% Fewer Hallucinations
Browse by: Latest US Patents, China's latest patents, Technical Efficacy Thesaurus, Application Domain, Technology Topic, Popular Technical Reports.
© 2025 PatSnap. All rights reserved.Legal|Privacy policy|Modern Slavery Act Transparency Statement|Sitemap|About US| Contact US: help@patsnap.com