A Risk-Aware Deep Learning-Driven Limit Transmission Capacity Adjustment Method
A technology of transmission capacity and deep learning, which is applied in the field of risk-aware deep learning-driven limit transmission capacity adjustment, which can solve problems such as the compatibility between machine learning methods and regression problems needs to be studied, it is difficult to ensure the economy of regulation, and the convergence is reduced.
- Summary
- Abstract
- Description
- Claims
- Application Information
AI Technical Summary
Problems solved by technology
Method used
Image
Examples
Embodiment Construction
[0051] The technical solutions of the present invention will be described in detail below with reference to the accompanying drawings, but the scope of the invention is not limited to the following.
[0052] like figure 1 As shown, in most OPF issues, the transfer capacity is often considered constant, and it is convenient to solve the model. However, as the operating conditions change, TTC will also change, which may result in security issues. In order to solve this problem, a feasible method is to use a double-layer model to solve, so that the TTC calculation model is used as the lower model, and the TTC security constraint is added to the upper economic scheduling model. Among them, TTC Safety Constraints are always greater than the cross-section transmission trend for the control section TTC. However, the convergence difficulties and computational burden of the lower TTC calculation model are solved, which makes it difficult to solve the TTC adjustment double-layer model. Ther...
PUM
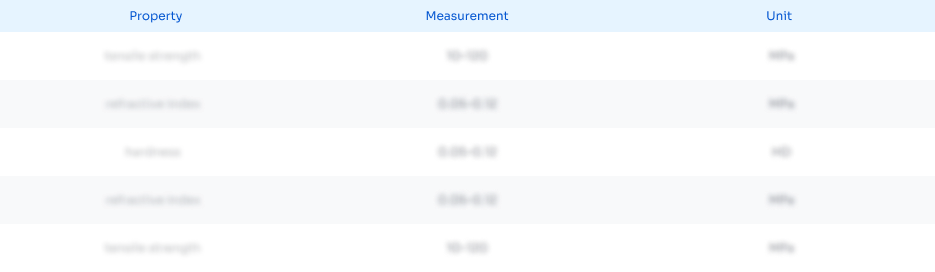
Abstract
Description
Claims
Application Information
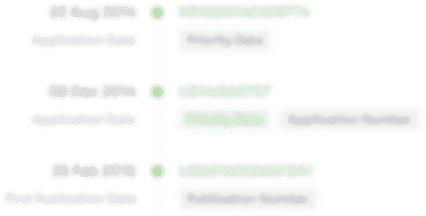
- Generate Ideas
- Intellectual Property
- Life Sciences
- Materials
- Tech Scout
- Unparalleled Data Quality
- Higher Quality Content
- 60% Fewer Hallucinations
Browse by: Latest US Patents, China's latest patents, Technical Efficacy Thesaurus, Application Domain, Technology Topic, Popular Technical Reports.
© 2025 PatSnap. All rights reserved.Legal|Privacy policy|Modern Slavery Act Transparency Statement|Sitemap|About US| Contact US: help@patsnap.com