Attention LSTM-based reinforcement learning Agent knowledge reasoning method
A technology of reinforcement learning and knowledge reasoning, applied in the fields of reinforcement learning and deep learning, it can solve problems such as inapplicability of large-scale knowledge graphs and ineffective memory, and achieve the effect of realizing memory path screening, suppressing invalid states, and optimizing the reward mechanism.
- Summary
- Abstract
- Description
- Claims
- Application Information
AI Technical Summary
Problems solved by technology
Method used
Image
Examples
Embodiment 1
[0032] The preprocessing operations in step a) include inference path information for statistical training, inference path information for statistical testing, and tokenization of entity relationships.
Embodiment 2
[0034] In step b), through the OpenKE-based TransH, TransE, TransR, DistinctMult, and CompLEx embedding models, the embedded word vector representation of the entity relationship in the triplet is obtained, and each entity and relationship is mapped to a dense continuous word vector.
Embodiment 3
[0036] In step c), through the formula P(S t+1 =s'|S t =s,max(A t )=a) Define the state transition equation at time t, where P is the probability of selecting a s' at time t+1, s' is the state variable at time t+1, and a is the state S at time t. t The maximum probability relation chosen, S t+1 is the state at time t+1, s is the entity associated with a, A t =P softmax (a|θ), θ is the network model parameter, through the formula Define the return function R(s t ), where e endThe final entity inferred for the relational path, e target target entity for relational path inference, e source is the set of entities in a given training path, e noanswer No node was found when reasoning the path, r + Indicates that the return value is a positive number, r - Indicates that the return value is negative.
PUM
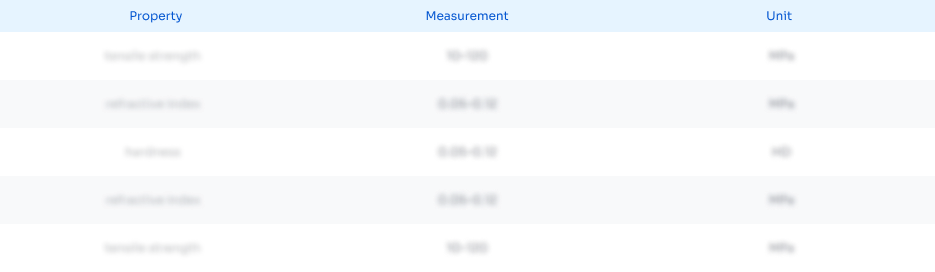
Abstract
Description
Claims
Application Information
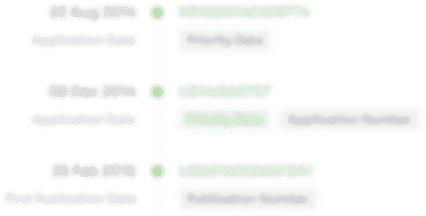
- R&D
- Intellectual Property
- Life Sciences
- Materials
- Tech Scout
- Unparalleled Data Quality
- Higher Quality Content
- 60% Fewer Hallucinations
Browse by: Latest US Patents, China's latest patents, Technical Efficacy Thesaurus, Application Domain, Technology Topic, Popular Technical Reports.
© 2025 PatSnap. All rights reserved.Legal|Privacy policy|Modern Slavery Act Transparency Statement|Sitemap|About US| Contact US: help@patsnap.com