Serialization task completion method and system based on memory consolidation mechanism and GAN model
A task completion and serialization technology, applied in neural learning methods, biological neural network models, computer components, etc., can solve problems such as inability to complete serialization tasks, model forgetting, etc.
- Summary
- Abstract
- Description
- Claims
- Application Information
AI Technical Summary
Problems solved by technology
Method used
Image
Examples
Embodiment approach
[0125] Here, as the first embodiment of the present invention, when it is the task of continuous image generation, the neural network often faces the situation of insufficient data volume during the training process. The insufficient data volume will cause the network to be unable to fully learn the characteristics of the data, resulting in Model overfitting problem. One way to solve the insufficient amount of data is to expand the data set, and using GAN to generate the data set is an effective and simple method. We apply the GAN continuous learning system to image generation and test whether it has the ability to continuously generate multi-category images. In the experiment, the MNIST dataset is used, which contains handwritten digits 0, 1, 2, 3, 4, 5, 6, 7, 8 and 9. We divide it into two groups, the first group is 0-4 and the second group is 5-9. Task 1 is to generate the first set of numbers and task 2 is to generate the second set of numbers. Specific steps are as fol...
PUM
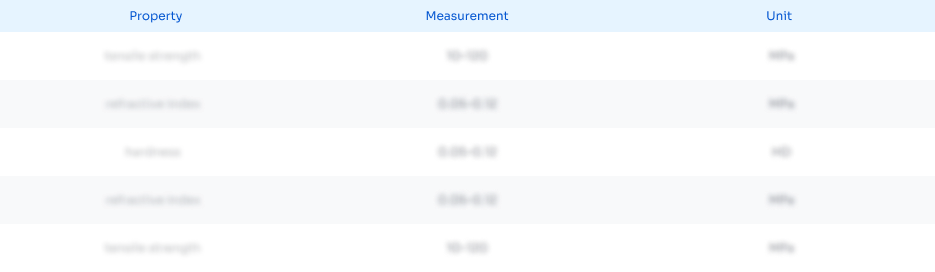
Abstract
Description
Claims
Application Information
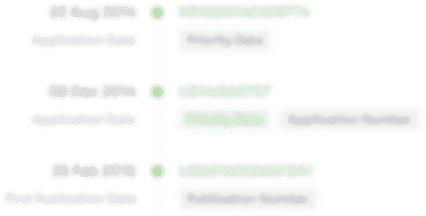
- R&D
- Intellectual Property
- Life Sciences
- Materials
- Tech Scout
- Unparalleled Data Quality
- Higher Quality Content
- 60% Fewer Hallucinations
Browse by: Latest US Patents, China's latest patents, Technical Efficacy Thesaurus, Application Domain, Technology Topic, Popular Technical Reports.
© 2025 PatSnap. All rights reserved.Legal|Privacy policy|Modern Slavery Act Transparency Statement|Sitemap|About US| Contact US: help@patsnap.com