Heterogeneous neural network knowledge recombination method based on common feature learning
A neural network and common feature technology, applied in the field of heterogeneous neural network knowledge reorganization, can solve infeasible problems, achieve the effect of learning robustness and saving labor costs
- Summary
- Abstract
- Description
- Claims
- Application Information
AI Technical Summary
Problems solved by technology
Method used
Image
Examples
Embodiment Construction
[0030] The experimental method of the present invention will be described in detail below in conjunction with the accompanying drawings and implementation examples, so as to fully understand and implement the process of how to apply technical means to solve technical problems and achieve technical effects in the present invention. It should be noted that, as long as there is no conflict, each embodiment of the present invention and each feature in each embodiment can be combined or out of sequence, and the formed technical solutions are within the protection scope of the present invention.
[0031] The heterogeneous neural network knowledge reorganization method based on common feature learning provided by the present invention, its specific framework is as follows figure 1 As shown, suppose there are N teacher networks, and each teacher network uses T i Indicates that the method includes:
[0032]Step 1, under the same input, align the output features of the teacher model an...
PUM
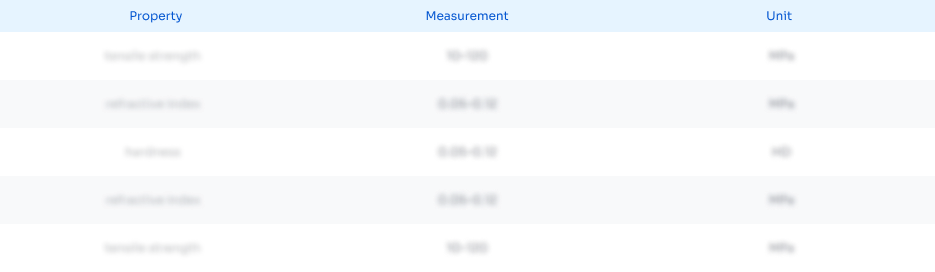
Abstract
Description
Claims
Application Information
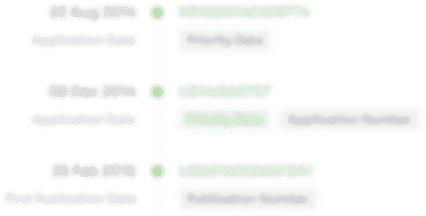
- R&D Engineer
- R&D Manager
- IP Professional
- Industry Leading Data Capabilities
- Powerful AI technology
- Patent DNA Extraction
Browse by: Latest US Patents, China's latest patents, Technical Efficacy Thesaurus, Application Domain, Technology Topic, Popular Technical Reports.
© 2024 PatSnap. All rights reserved.Legal|Privacy policy|Modern Slavery Act Transparency Statement|Sitemap|About US| Contact US: help@patsnap.com