A voiceprint recognition method based on self-attention and transfer learning
A transfer learning and voiceprint recognition technology, applied in speech analysis, instruments, etc., can solve the problems of lack of generalization ability of real-world applications, low accuracy of voiceprint recognition, etc., to expand generalization ability, strengthen generalization ability, high precision effect
- Summary
- Abstract
- Description
- Claims
- Application Information
AI Technical Summary
Problems solved by technology
Method used
Image
Examples
Embodiment 1
[0029] A voiceprint recognition method based on self-attention and transfer learning, which obtains open-source English speech data and constructs a first-level basic data set; obtains open-source Chinese speech data and constructs a second-level basic data set; collects voice data of application scenarios and constructs application scenarios dataset; such as Figure 6 As shown, based on the attention model and the first-level basic data set, the first-level basic model is trained; then, on the second-level basic data set, the first-level basic model is migrated and fine-tuned to obtain the second-level basic model; finally, in the specific Based on the application scenario data, migrate and fine-tune the secondary basic model to obtain the final model suitable for the specific application scenario. Cascade fine-tuning not only learns the robustness of noise, reverberation, and channels, but also learns the pronunciation characteristics of Chinese and the recognition ability t...
Embodiment 2
[0032] This embodiment optimizes on the basis of embodiment 1, obtains massive open-source English voice data (sitw, voxceleb1, voxceleb2, etc.), and builds a first-level voiceprint basic data set; this data set is collected under unconstrained conditions and has a large Good noise, reverberation, channel robustness.
[0033] Obtain a large amount of open source Chinese speech data (aishell, primewords, st-cmds, thchs30, etc.), and construct a secondary voiceprint basic data set; this data set is a Chinese data set, which can better adapt to the pronunciation characteristics of Chinese.
[0034] Collect a small amount of voice data in application scenarios to build an application scenario voiceprint data set; this data set is collected in real application scenarios, which can better match the actual application scenarios.
[0035] Other parts of this embodiment are the same as those of Embodiment 1, so details are not repeated here.
Embodiment 3
[0037] This embodiment is optimized on the basis of embodiment 1 or 2, as figure 1 , figure 2 As shown, data enhancement in the time domain and frequency domain is performed on the first-level basic data set, the second-level basic data set, and the application scenario data set. like figure 1 As shown, the time domain audio data is enhanced; in the time domain, the rhythm and pitch are controlled, the audio speed is adjusted, and random noise is added. like figure 2 As shown, the audio data in the frequency domain is enhanced; in the frequency domain, Vocal Tract Length Perturbation is used to apply a random distortion factor to the spectral characteristics of each audio.
[0038] The invention obtains English and Chinese public data sets, collects a small amount of application scene data sets, and enhances them from two dimensions of time domain and frequency domain. For all data sets, data enhancement in the time domain and frequency domain is carried out, which great...
PUM
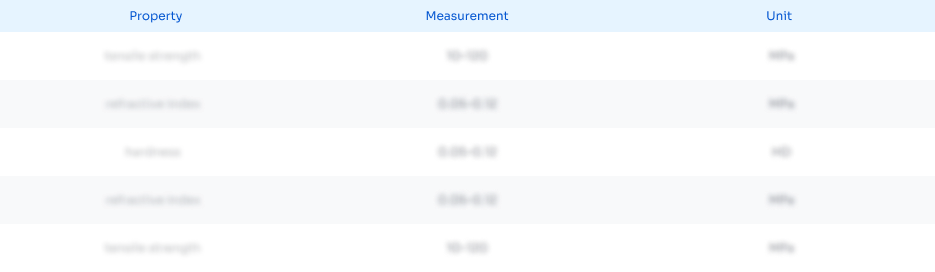
Abstract
Description
Claims
Application Information
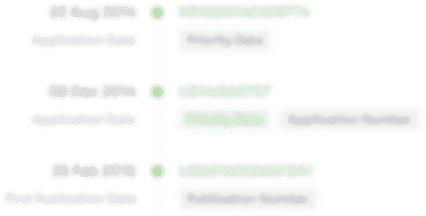
- R&D
- Intellectual Property
- Life Sciences
- Materials
- Tech Scout
- Unparalleled Data Quality
- Higher Quality Content
- 60% Fewer Hallucinations
Browse by: Latest US Patents, China's latest patents, Technical Efficacy Thesaurus, Application Domain, Technology Topic, Popular Technical Reports.
© 2025 PatSnap. All rights reserved.Legal|Privacy policy|Modern Slavery Act Transparency Statement|Sitemap|About US| Contact US: help@patsnap.com