Image Classification Method Based on Denoising Sparse Autoencoder and Density Spatial Sampling
A sparse autoencoder, spatial sampling technology, used in instrumentation, character and pattern recognition, computing, etc.
- Summary
- Abstract
- Description
- Claims
- Application Information
AI Technical Summary
Problems solved by technology
Method used
Image
Examples
Embodiment Construction
[0042] The technical solutions of the present invention will be described in detail below in conjunction with the accompanying drawings.
[0043] The test experiment hardware and software environment of the present embodiment is as follows:
[0044] Hardware type:
[0045] Computer type: desktop;
[0046] CPU: Intel(R) Core(TM) i5-5200U CPU@2.20GHz
[0047] Memory: 8.00GB
[0048] System type: 64-bit operating system
[0049] Development language: Matlab
[0050] The technical solutions of the present invention will be described in detail below in conjunction with the accompanying drawings. The embodiment takes the STL-10 database as an example, the database contains 10 types of RGB images, and the size of each image is 96*96. The number of training samples used for supervised training is 5000 in total, and the 5000 training samples are divided into ten folds. The number of training samples used for supervised training each time is 1000, and the number of test samples is...
PUM
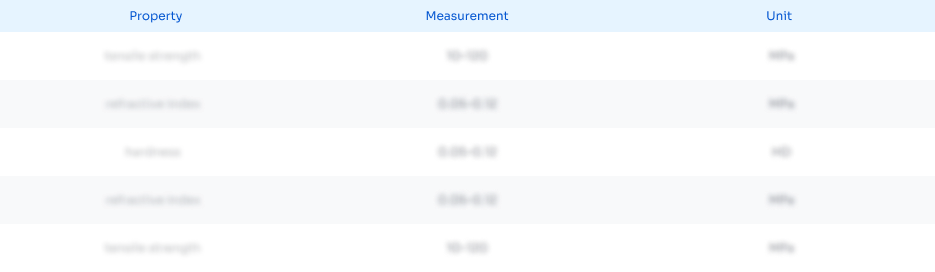
Abstract
Description
Claims
Application Information
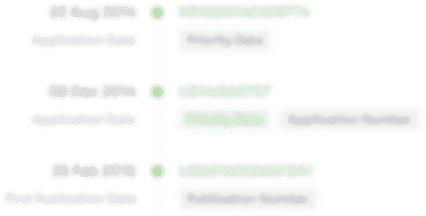
- R&D Engineer
- R&D Manager
- IP Professional
- Industry Leading Data Capabilities
- Powerful AI technology
- Patent DNA Extraction
Browse by: Latest US Patents, China's latest patents, Technical Efficacy Thesaurus, Application Domain, Technology Topic.
© 2024 PatSnap. All rights reserved.Legal|Privacy policy|Modern Slavery Act Transparency Statement|Sitemap