Convolutional neural network emotion image classification method combining emotion category attention loss
A convolutional neural network and attention technology, applied in the field of emotional image classification of convolutional neural network combined with emotional category attention loss, can solve problems such as uniform distribution by category, no loss function design, and the number of emotional images cannot be achieved. , to achieve a good emotional classification effect and reduce the possible effect of misclassification
- Summary
- Abstract
- Description
- Claims
- Application Information
AI Technical Summary
Problems solved by technology
Method used
Image
Examples
Embodiment Construction
[0018] The present invention proposes a convolutional neural network emotional image classification method combined with emotional category attention loss. Overall structure of the present invention is as figure 1 shown. The example of the present invention is simulated under the environment of win10 and JupyterNotebook, and the FI data set is used for training through the method of the present invention to obtain an image emotion classification model that can achieve high accuracy. After the model is obtained, the test image can be input into the model to obtain the sentiment classification result of the image. The concrete realization process of the present invention is as figure 2 As shown, the specific implementation steps are as follows:
[0019] Step 1: set up the emotion category weight vector of image: organize and divide the emotion image data set with label, regard the image of each class in the training set part as one-dimensional, obtain emotion category weight...
PUM
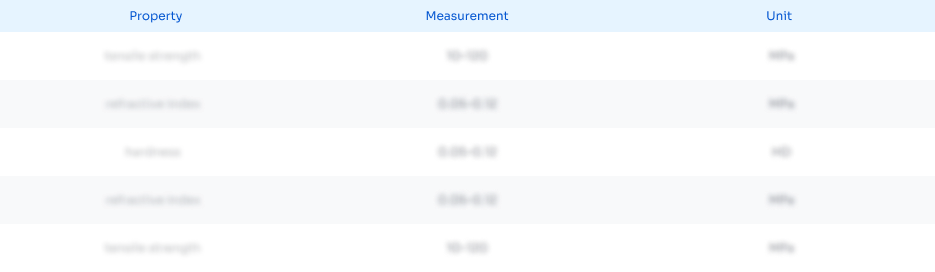
Abstract
Description
Claims
Application Information
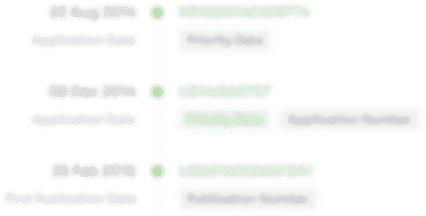
- R&D Engineer
- R&D Manager
- IP Professional
- Industry Leading Data Capabilities
- Powerful AI technology
- Patent DNA Extraction
Browse by: Latest US Patents, China's latest patents, Technical Efficacy Thesaurus, Application Domain, Technology Topic, Popular Technical Reports.
© 2024 PatSnap. All rights reserved.Legal|Privacy policy|Modern Slavery Act Transparency Statement|Sitemap|About US| Contact US: help@patsnap.com