Multi-view stereoscopic vision three-dimensional scene reconstruction method based on deep learning
A technology of stereo vision and 3D scene, applied in the field of computer vision and 3D reconstruction, to achieve the effect of high-precision 3D scene reconstruction
- Summary
- Abstract
- Description
- Claims
- Application Information
AI Technical Summary
Problems solved by technology
Method used
Image
Examples
Embodiment Construction
[0018] The specific process of the present invention will be described in detail below:
[0019] 1. Multi-scale feature extraction and fusion
[0020] This part is mainly to extract the multi-scale features of the image and the aggregation of multi-scale feature bodies. Its innovation is to propose a multi-scale feature volume aggregation network, namely MFVA-Net (Multi-scale Feature Volume AggregationNet), which can learn context information in different scale feature volumes and enhance the ability of neural networks to predict depth. Further improve the accuracy and integrity of 3D reconstruction.
[0021] The multi-scale feature extraction and fusion part mainly consists of three stages: 1) multi-scale feature extraction; 2) construction of feature volume; 3) aggregation of multi-scale feature volume. Its frame as figure 2 .
[0022] 1) Multi-scale feature extraction
[0023] The input of the network is N RGB images with known camera parameters Will I 1 Remember as...
PUM
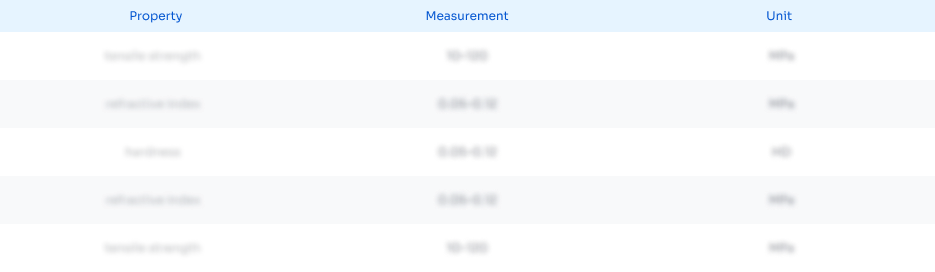
Abstract
Description
Claims
Application Information
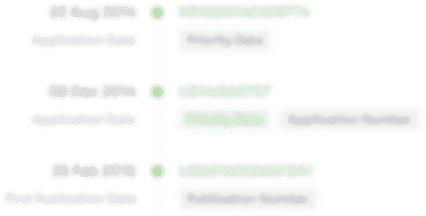
- R&D Engineer
- R&D Manager
- IP Professional
- Industry Leading Data Capabilities
- Powerful AI technology
- Patent DNA Extraction
Browse by: Latest US Patents, China's latest patents, Technical Efficacy Thesaurus, Application Domain, Technology Topic, Popular Technical Reports.
© 2024 PatSnap. All rights reserved.Legal|Privacy policy|Modern Slavery Act Transparency Statement|Sitemap|About US| Contact US: help@patsnap.com