Depth-first data scheduling method, system and equipment based on block convolution
A data scheduling and depth-first technology, applied in the field of convolutional neural networks, can solve problems such as unsuitable deployment and image processing occupying too much memory, so as to avoid memory consumption and improve reasoning efficiency
- Summary
- Abstract
- Description
- Claims
- Application Information
AI Technical Summary
Problems solved by technology
Method used
Image
Examples
Embodiment Construction
[0034] The application will be further described in detail below in conjunction with the accompanying drawings and embodiments. It should be understood that the specific embodiments described here are only used to explain related inventions, not to limit the invention. It should also be noted that, for the convenience of description, only the parts related to the related invention are shown in the drawings.
[0035] It should be noted that, in the case of no conflict, the embodiments in the present application and the features in the embodiments can be combined with each other. The present application will be described in detail below with reference to the accompanying drawings and embodiments.
[0036] A block convolution-based depth-first data scheduling method of the present invention includes:
[0037] Step S100, divide the 0th layer feature map feature0 into m*n blocks with a preset size of B, and set the coordinate index (X, Y), initialize (X, Y) = (0, 0), feature map ...
PUM
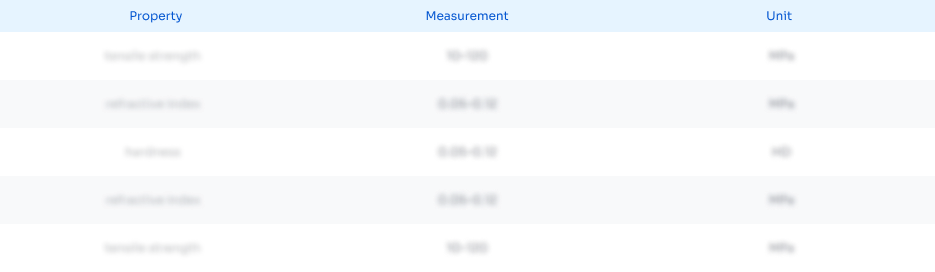
Abstract
Description
Claims
Application Information
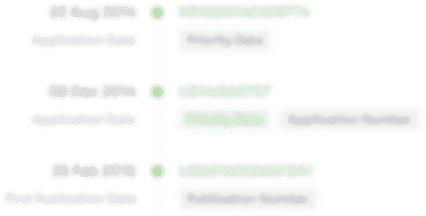
- R&D Engineer
- R&D Manager
- IP Professional
- Industry Leading Data Capabilities
- Powerful AI technology
- Patent DNA Extraction
Browse by: Latest US Patents, China's latest patents, Technical Efficacy Thesaurus, Application Domain, Technology Topic.
© 2024 PatSnap. All rights reserved.Legal|Privacy policy|Modern Slavery Act Transparency Statement|Sitemap