A cross-modal image-text association anomaly detection method
An anomaly detection and cross-modal technology, applied in the direction of neural learning methods, character and pattern recognition, biological neural network models, etc., can solve the problem that it is difficult to fully learn the data association of different modalities, and it is difficult to establish data of different modalities Contact and other issues to achieve the effect of improving accuracy and robustness
- Summary
- Abstract
- Description
- Claims
- Application Information
AI Technical Summary
Problems solved by technology
Method used
Image
Examples
Embodiment 1
[0027] The present invention provides a cross-modal image-text correlation anomaly detection method, the flowchart of which is as follows figure 1 As shown, it is divided into three stages, namely the image multi-label classification stage, the text multi-label classification stage and the associated anomaly detection stage. details as follows:
[0028] 1. Image multi-label classification stage
[0029] The input of the image multi-label classification stage is the image in the image-text pair to be detected. The image multi-label classification model consists of a CNN encoder and an RNN decoder. The CNN encoder is used to extract important visual features from the image, and then the extracted The features of the input RNN decoder generate a sequence of labels to predict the final label of the image. First, the image is preprocessed. In the preprocessing stage, the size of the image is adjusted to a fixed shape of 288*288, and then the pixels of the three channels of the i...
PUM
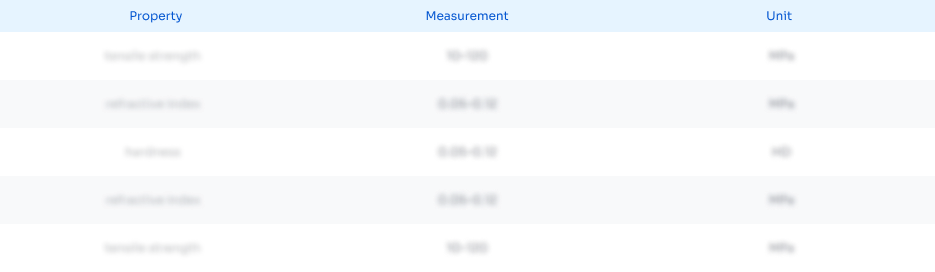
Abstract
Description
Claims
Application Information
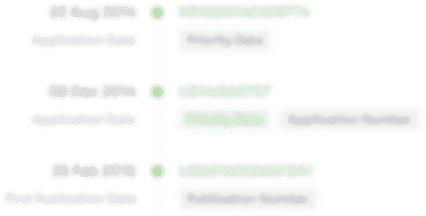
- R&D Engineer
- R&D Manager
- IP Professional
- Industry Leading Data Capabilities
- Powerful AI technology
- Patent DNA Extraction
Browse by: Latest US Patents, China's latest patents, Technical Efficacy Thesaurus, Application Domain, Technology Topic.
© 2024 PatSnap. All rights reserved.Legal|Privacy policy|Modern Slavery Act Transparency Statement|Sitemap