Depth decoupling time sequence prediction method
A technology of time series and forecasting methods, applied in neural learning methods, neural architectures, biological neural network models, etc., can solve problems such as the inability to capture global change patterns, and achieve the effect of improving forecasting accuracy
- Summary
- Abstract
- Description
- Claims
- Application Information
AI Technical Summary
Problems solved by technology
Method used
Image
Examples
Embodiment Construction
[0028] In order to make the object, technical solution and advantages of the present invention clearer, the present invention will be further described in detail below in conjunction with the accompanying drawings and embodiments. It should be understood that the specific embodiments described here are only used to explain the present invention, and do not limit the protection scope of the present invention.
[0029] In order to improve the forecasting accuracy of time series, the embodiment provides a deep decoupling time series forecasting method, by decoupling the dynamics of time series into a global change mode and a local change mode, and modeling them separately, based on the two changes model for time series forecasting. This deep decoupling time series prediction method can be applied to the fields of transportation, electric power, medical treatment and finance, that is, the time series can be data such as road traffic flow, user electricity consumption and stock pri...
PUM
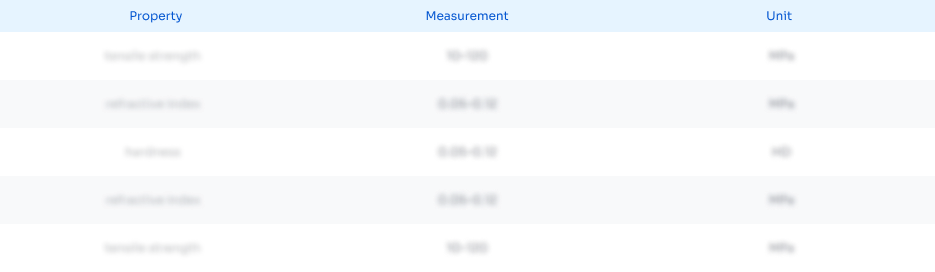
Abstract
Description
Claims
Application Information
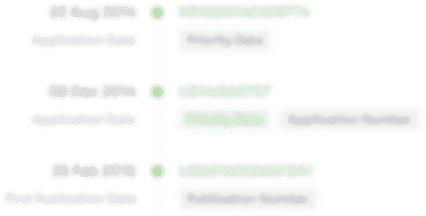
- R&D
- Intellectual Property
- Life Sciences
- Materials
- Tech Scout
- Unparalleled Data Quality
- Higher Quality Content
- 60% Fewer Hallucinations
Browse by: Latest US Patents, China's latest patents, Technical Efficacy Thesaurus, Application Domain, Technology Topic, Popular Technical Reports.
© 2025 PatSnap. All rights reserved.Legal|Privacy policy|Modern Slavery Act Transparency Statement|Sitemap|About US| Contact US: help@patsnap.com