Pedestrian re-recognition system and method based on spatial sequence feature learning
A pedestrian re-identification and spatial sequence technology, applied in the field of pedestrian re-identification, can solve problems such as model interference, cumbersome process, and increased algorithm complexity
- Summary
- Abstract
- Description
- Claims
- Application Information
AI Technical Summary
Problems solved by technology
Method used
Image
Examples
Embodiment 1
[0044] The network framework used in the present invention is as figure 1As shown, the triplet image is used as input, and the Res2Net-50 network is used for feature extraction, and the feature map extracted by stage4 is input into the global feature branch and the spatial sequence feature learning branch respectively. In the global feature branch, the feature vector is first reduced in dimension through the average pooling operation, and then input to the fully connected layer to map to the classification space, and the Ranked List Loss and AM-Softmax Loss are calculated. In the spatial sequence feature learning branch, the dimensionality is first reduced to 1024 through a 1*1 convolutional layer, and then a random mask is used to suppress some areas of the feature map, and then maximum pooling is performed in the row and column directions to obtain different spatial Dimensionally eigenvectors. Then input them into the self-attention module to learn the spatial sequence feat...
Embodiment 2
[0087] Experimental setup:
[0088] Experimental environment: The code is written using the Pytorch framework and runs on a server configured with two Nvidia TITAN Xp graphics cards.
[0089] Res2Net: The backbone network uses the Res2Net-50 network pre-trained on ImageNet. Its structure is similar to Res2Net-50. Only the residual module is replaced, and the number of sub-feature maps is s=4. The size of the final output feature map is 16*8*2048.
[0090] Spatial sequence feature learning module: self-attention module part, the number of modules is N=4, the module dimension in a single module is d=1024, and the number of multi-head attention heads is h=8. And the random mask part, R h Choose randomly within the set {0, 0.1, 0.2, 0.3}, R w =1.
[0091] GAN network:
[0092] Since the GAN network only generates images, it is necessary to perform data enhancement in the pedestrian recognition model. The present invention uses the Densenet-121 network as the baseline of the ...
PUM
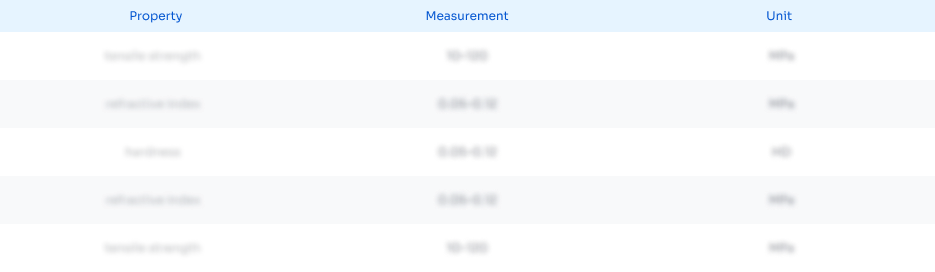
Abstract
Description
Claims
Application Information
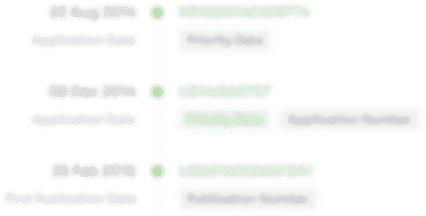
- R&D Engineer
- R&D Manager
- IP Professional
- Industry Leading Data Capabilities
- Powerful AI technology
- Patent DNA Extraction
Browse by: Latest US Patents, China's latest patents, Technical Efficacy Thesaurus, Application Domain, Technology Topic, Popular Technical Reports.
© 2024 PatSnap. All rights reserved.Legal|Privacy policy|Modern Slavery Act Transparency Statement|Sitemap|About US| Contact US: help@patsnap.com