Aspect-level text sentiment analysis method based on heterogeneous graph neural network
A neural network and sentiment analysis technology, applied in neural learning methods, biological neural network models, semantic analysis, etc., can solve the problems of poor model generalization ability, model performance decline, loss of information, etc., to improve expression ability and generalization. The effect of the ability to
- Summary
- Abstract
- Description
- Claims
- Application Information
AI Technical Summary
Problems solved by technology
Method used
Image
Examples
Embodiment Construction
[0037]In order to better understand the present invention, the technical solutions in the embodiments of the present invention will be described in connection with the drawings in the embodiments of the present invention, and the embodiments described herein will be clearly understood. It is an embodiment of the invention, not all of the embodiments. Based on the embodiments in the present invention, those of ordinary skill in the art will belong to the scope of the invention in the present invention without making in the pre-creative labor premise.
[0038] It should be noted that the specification and claims of the present invention and the terms "first", "second", "second" or the like are used to distinguish a similar object without having to describe a particular order or ahead order. It should be understood that the data such as use can be interchanged in place so that the embodiments of the invention described herein can be implemented in the order other than those illustrat...
PUM
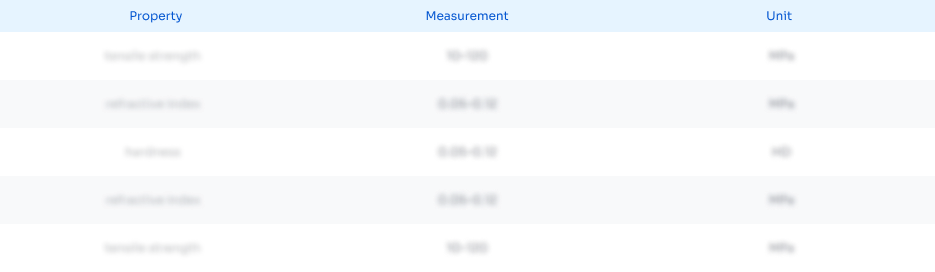
Abstract
Description
Claims
Application Information
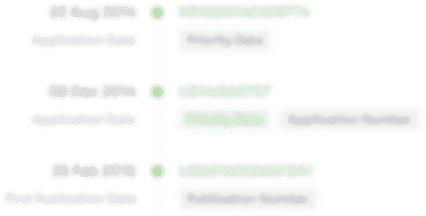
- R&D Engineer
- R&D Manager
- IP Professional
- Industry Leading Data Capabilities
- Powerful AI technology
- Patent DNA Extraction
Browse by: Latest US Patents, China's latest patents, Technical Efficacy Thesaurus, Application Domain, Technology Topic, Popular Technical Reports.
© 2024 PatSnap. All rights reserved.Legal|Privacy policy|Modern Slavery Act Transparency Statement|Sitemap|About US| Contact US: help@patsnap.com