Data denoising method based on mark risk control
A risk control and data technology, applied in neural learning methods, neural architectures, biological neural network models, etc., can solve the problems of difficulty in collecting data, consuming a lot of manpower and material resources, etc., achieving strong robustness, good image noise removal, and prevention. The effect of learning performance degradation
- Summary
- Abstract
- Description
- Claims
- Application Information
AI Technical Summary
Problems solved by technology
Method used
Image
Examples
Embodiment Construction
[0013] Below in conjunction with specific embodiment, further illustrate the present invention, should be understood that these embodiments are only used to illustrate the present invention and are not intended to limit the scope of the present invention, after having read the present invention, those skilled in the art will understand various equivalent forms of the present invention All modifications fall within the scope defined by the appended claims of the present application.
[0014] Such as figure 1 As shown, the data denoising method based on labeling risk control maintains two neural networks based on the small loss criterion and selects data with small loss as low-risk data to update the peer-to-peer network, and each network finds and removes high-risk data. And retrain on the remaining data, pay attention to the inconsistency of the two networks during the training process, if the inconsistency tends to be stable or the number of learning rounds reaches the preset...
PUM
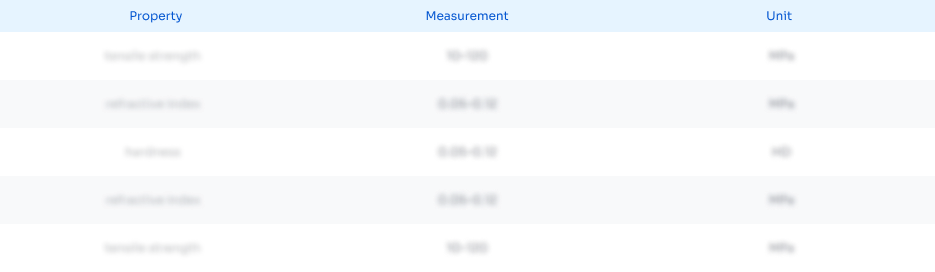
Abstract
Description
Claims
Application Information
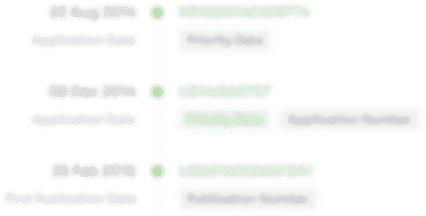
- Generate Ideas
- Intellectual Property
- Life Sciences
- Materials
- Tech Scout
- Unparalleled Data Quality
- Higher Quality Content
- 60% Fewer Hallucinations
Browse by: Latest US Patents, China's latest patents, Technical Efficacy Thesaurus, Application Domain, Technology Topic, Popular Technical Reports.
© 2025 PatSnap. All rights reserved.Legal|Privacy policy|Modern Slavery Act Transparency Statement|Sitemap|About US| Contact US: help@patsnap.com