Multi-label image classification method and system based on class activation mapping mechanism
A technology of mapping mechanism and classification method, applied in computer parts, character and pattern recognition, instruments, etc., can solve problems such as affecting the image recognition performance of graphic classification models, not considering image visual consistency, etc., to maintain visual consistency, The effect of improving the classification effect
- Summary
- Abstract
- Description
- Claims
- Application Information
AI Technical Summary
Problems solved by technology
Method used
Image
Examples
Embodiment Construction
[0068] In order to make the purpose, technical solution and advantages of the present application clearer, the present application will be further described in detail below in conjunction with the accompanying drawings and embodiments. It should be understood that the specific embodiments described here are only used to explain the present application, and are not intended to limit the present application.
[0069] Aiming at the problems existing in the prior art, an embodiment of the present invention provides a multi-label image classification method based on a class activation mapping mechanism, including:
[0070] Obtain an image to be classified, and convert the image to be classified into a multidimensional tensor;
[0071] Input the multidimensional tensor into the classification model to obtain the classification result of the image to be classified;
[0072] Wherein, the classification model is obtained through the following steps of training:
[0073] Obtain a trai...
PUM
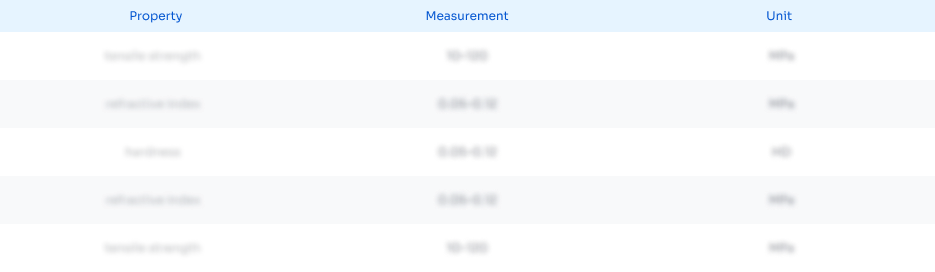
Abstract
Description
Claims
Application Information
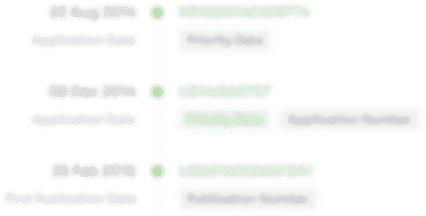
- R&D Engineer
- R&D Manager
- IP Professional
- Industry Leading Data Capabilities
- Powerful AI technology
- Patent DNA Extraction
Browse by: Latest US Patents, China's latest patents, Technical Efficacy Thesaurus, Application Domain, Technology Topic, Popular Technical Reports.
© 2024 PatSnap. All rights reserved.Legal|Privacy policy|Modern Slavery Act Transparency Statement|Sitemap|About US| Contact US: help@patsnap.com