Multi-label image classification method based on multi-scale and cross-modal attention mechanism
A classification method and multi-scale technology, applied in neural learning methods, computer components, character and pattern recognition, etc., can solve the limitation of feature extraction, insufficient utilization of label features and image features, and the fusion angle of image features and label features Single and other problems, to increase the angle of information utilization, improve the effect of prediction, and increase the effect of richness
- Summary
- Abstract
- Description
- Claims
- Application Information
AI Technical Summary
Problems solved by technology
Method used
Image
Examples
Embodiment
[0072] A multi-label image classification method based on multi-scale and cross-modal attention mechanisms, such as figure 1 , figure 2 shown, including the following steps:
[0073] S1. Construct a label map and learn label features through a graph convolutional neural network;
[0074] In this embodiment, the first training set MS-COCO is obtained, the number of occurrences of various labels in the first training set is counted, and the conditional probability between any two types of labels is calculated according to the number of occurrences of each type of label in the first training set, All conditional probabilities form a relationship matrix A, and the obtained tag word vector matrix H and relationship matrix A are input into the graph convolutional neural network (GCN) to obtain the co-occurrence relationship word vector matrix W corresponding to all C-type tags.
[0075] Step S1 specifically includes the following steps:
[0076] S1.1. Count the number of occurre...
PUM
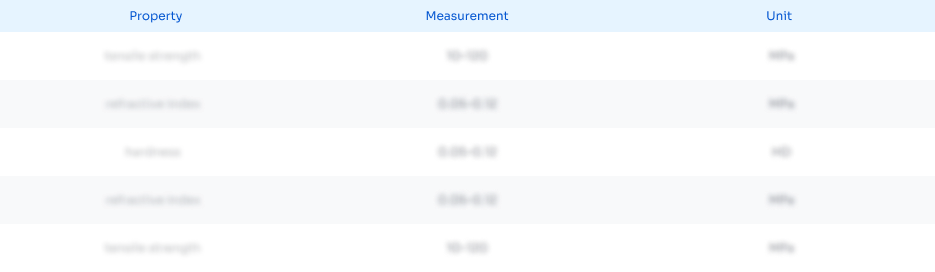
Abstract
Description
Claims
Application Information
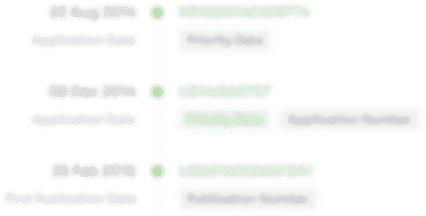
- R&D Engineer
- R&D Manager
- IP Professional
- Industry Leading Data Capabilities
- Powerful AI technology
- Patent DNA Extraction
Browse by: Latest US Patents, China's latest patents, Technical Efficacy Thesaurus, Application Domain, Technology Topic, Popular Technical Reports.
© 2024 PatSnap. All rights reserved.Legal|Privacy policy|Modern Slavery Act Transparency Statement|Sitemap|About US| Contact US: help@patsnap.com