Federal learning member reasoning attack defense method based on adversarial interference
An adversarial and member-based technology, applied in machine learning, electrical digital data processing, biological neural network models, etc., can solve problems such as member reasoning attacks, leakage of user local data privacy, etc., and achieve the effect of reducing the loss of accuracy
- Summary
- Abstract
- Description
- Claims
- Application Information
AI Technical Summary
Problems solved by technology
Method used
Image
Examples
Embodiment 1
[0059] In this embodiment, a federated learning member reasoning attack defense model based on an adversarial interference-based federated learning member reasoning attack defense method is established, as shown in figure 1 shown.
[0060] figure 1 The following federated learning membership inference attack defense scenarios are described.
[0061] There are 100 participants and 1 server in this scenario; the participants train the local model and upload parameters, and the server aggregates the parameters and updates the global model as one round of the federated learning training process. A total of 100 federated learnings are performed in this scenario Rounds; each participant iterates 5 rounds each time the local model is trained; set the federated learning parameters batchsize=128, learning rate=0.1, momentum=0.9, milestones=[60,90], where batch size is batch The processing size refers to the amount of data in one training session when the participants train the local ...
Embodiment 2
[0078] In this embodiment, the results of the method of the present invention are compared in various scenarios, and it is verified that the defense method of the present invention is applicable to various data sets and target model structures. For the Purchase100 dataset, use a fully connected neural network as the target model; for the CIFAR10 dataset (http: / / www.cs.toronto.edu / ~kriz / cifar.html), use the AlexNet model for image classification as the target model ; For the CIFAR100 dataset (http: / / www.cs.toronto.edu / ~kriz / cifar.html), the AlexNet model and the DenseNet12 model are respectively used as the target model, and DenseNet12 corresponds to DenseNet-BC (depth L=100, each The number of feature maps output by each network layer k=12); when using different data sets and target model structures for undefended (skip the noise optimization part of step 2 and step 3) and defensive federated learning model training, due to The limit of the Cifar100 data set needs to set the n...
Embodiment 3
[0084] This embodiment compares the method described in the present invention with various federal learning member reasoning attack defense methods, and verifies that the defense method of the present invention has a better member reasoning attack defense effect than other defense methods and can maintain a lower performance loss.
[0085] Use Cifar10 as data set and AlexNet model as target model; The target model that does not use defense method training to obtain has 79% attack accuracy rate, 95% training accuracy rate, 0.1% training loss; Defense method proposed by the present invention has 53% attack accuracy, 95% training accuracy, 0.1% training loss.
[0086]The first defense method compared (https: / / dl.acm.org / doi / abs / 10.1145 / 3243734.3243855) is a federated learning member reasoning attack defense method based on adversarial regularization Ad-reg; set Ad-reg Adversarial regularization factor λ=2, Ad-reg has 59% attack accuracy, 99% training accuracy and 0.5% training l...
PUM
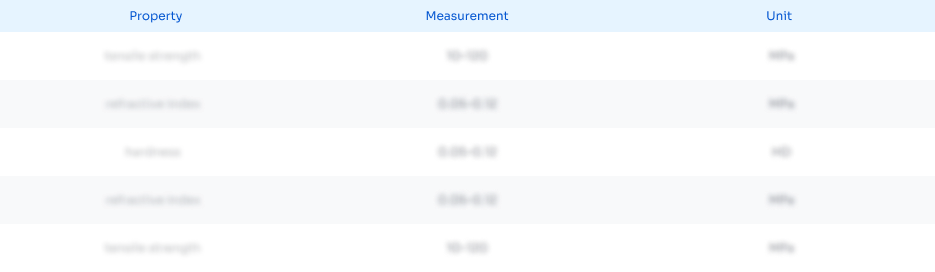
Abstract
Description
Claims
Application Information
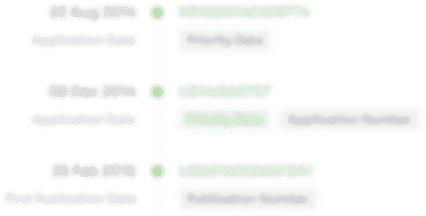
- R&D Engineer
- R&D Manager
- IP Professional
- Industry Leading Data Capabilities
- Powerful AI technology
- Patent DNA Extraction
Browse by: Latest US Patents, China's latest patents, Technical Efficacy Thesaurus, Application Domain, Technology Topic, Popular Technical Reports.
© 2024 PatSnap. All rights reserved.Legal|Privacy policy|Modern Slavery Act Transparency Statement|Sitemap|About US| Contact US: help@patsnap.com