Liver CT image segmentation system and algorithm based on mixed supervised learning
A CT image and supervised learning technology, applied in the interdisciplinary field of medicine and computer science, can solve a lot of time and labor costs, reduce manual labeling costs, and limited improvement, to improve the consistency of results, reduce the number of independent parameters, good consistency effect
- Summary
- Abstract
- Description
- Claims
- Application Information
AI Technical Summary
Problems solved by technology
Method used
Image
Examples
Embodiment Construction
[0034] The invention discloses a liver CT image segmentation system based on hybrid supervised learning. The segmentation system includes an image preprocessing unit, a feature extraction unit, a word vector segmentation unit and a single-layer convolution classification unit. The image segmentation system includes an image preprocessing unit, A feature extraction unit, a word vector segmentation unit and a single-layer convolution classification unit, the image preprocessing unit is connected to the feature extraction unit, and the feature extraction unit is respectively connected to the word vector segmentation unit and the single-layer convolution classification unit Make a data connection.
[0035] The construction process of the image preprocessing unit is:
[0036] The range of HU values in truncated CT slice images is [H 1 ,H 2 ], where H 1 and H 2 Indicates the lower and upper bounds of the rough HU value that can preserve the liver tissue intact and remove the b...
PUM
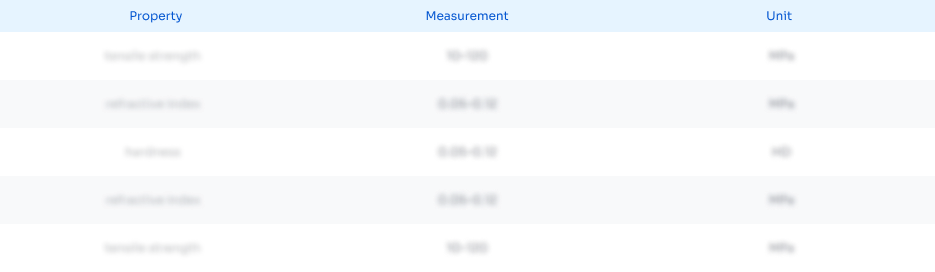
Abstract
Description
Claims
Application Information
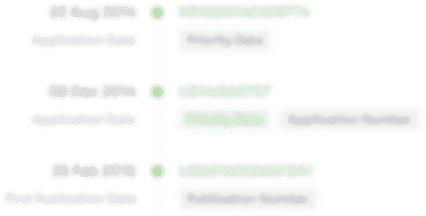
- R&D Engineer
- R&D Manager
- IP Professional
- Industry Leading Data Capabilities
- Powerful AI technology
- Patent DNA Extraction
Browse by: Latest US Patents, China's latest patents, Technical Efficacy Thesaurus, Application Domain, Technology Topic, Popular Technical Reports.
© 2024 PatSnap. All rights reserved.Legal|Privacy policy|Modern Slavery Act Transparency Statement|Sitemap|About US| Contact US: help@patsnap.com