Bearing fault diagnosis method based on prototype network
A prototype network and fault diagnosis technology, applied in neural learning methods, biological neural network models, instruments, etc., can solve problems such as expensive, prone to overfitting, and difficult cost of labeled data samples, achieving high accuracy and application Foreground-enhancing effect
- Summary
- Abstract
- Description
- Claims
- Application Information
AI Technical Summary
Problems solved by technology
Method used
Image
Examples
Embodiment 1
[0060] refer to figure 1 , the present invention provides a bearing fault diagnosis method based on a prototype network, comprising the following steps: obtaining a plurality of sample bearing vibration signals, dividing them into a support set and a query set; obtaining the support set and the query set by fast Fourier transform The frequency spectrum of the bearing vibration signal; using the deep neural network as the measurement module; using the convolution kernel from large to small as the feature extraction module; using the measurement module and the feature extraction module to build a prototype network model for bearing fault diagnosis; combining the support set and query set The spectrum data of the prototype network model is used as input, and the network parameters are obtained by obtaining the relationship score between the query set sample and the different types of class prototypes in the support set and the loss function value of the prototype network model thr...
PUM
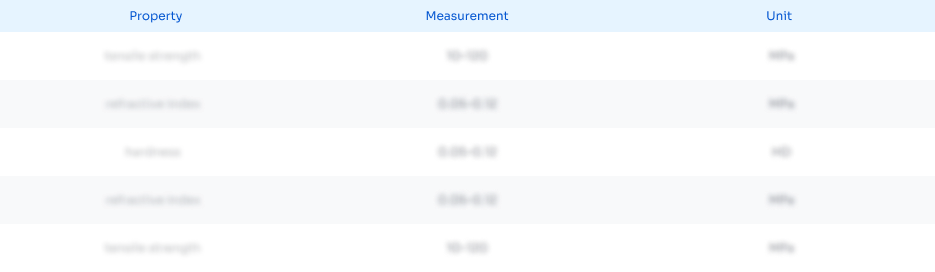
Abstract
Description
Claims
Application Information
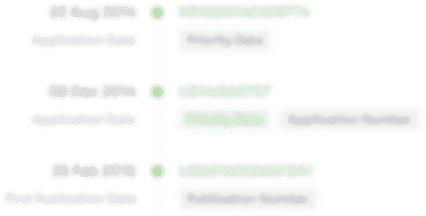
- R&D
- Intellectual Property
- Life Sciences
- Materials
- Tech Scout
- Unparalleled Data Quality
- Higher Quality Content
- 60% Fewer Hallucinations
Browse by: Latest US Patents, China's latest patents, Technical Efficacy Thesaurus, Application Domain, Technology Topic, Popular Technical Reports.
© 2025 PatSnap. All rights reserved.Legal|Privacy policy|Modern Slavery Act Transparency Statement|Sitemap|About US| Contact US: help@patsnap.com