Wavelet modified Bayesian convolution energy-based underwater vehicle propeller fault diagnosis method
A technology of underwater robots and propellers, which is applied in the direction of instruments, electrical testing/monitoring, control/regulation systems, etc. It can solve the problem of weak amplitude of dynamic signal singular behavior, affecting the identification of fault energy area boundaries, affecting the size of fault energy characteristics, etc. question
- Summary
- Abstract
- Description
- Claims
- Application Information
AI Technical Summary
Problems solved by technology
Method used
Image
Examples
Embodiment 1
[0055] like figure 1 As shown, a kind of underwater robot thruster fault diagnosis method based on wavelet modified Bayesian convolution energy of the present embodiment, the specific steps are as follows:
[0056] The first step is to collect and record dynamic signals such as the speed of the underwater robot and the rate of change of the thruster control voltage, using a length L 1 The time domain window function of =400 intercepts the dynamic signal;
[0057] In the second step, the conventional wavelet decomposition is performed on the data obtained in the first step to obtain the wavelet approximate component s A (n), n is the data serial number, and the value range of n is 1~L 1 Integer between;
[0058] In the third step, the conventional modified Bayesian algorithm is used to approximate the wavelet component s obtained in the second step A (n) to perform operations, wherein the modified Bayesian step size is N 2 , the calculation formulas are shown in formula (1...
Embodiment 2
[0088] like figure 2 As shown, a kind of underwater robot thruster fault diagnosis method based on wavelet modified Bayesian convolution energy of the present embodiment, the specific implementation steps are as follows:
[0089] The first step is to collect and record dynamic signals such as the speed signal of the underwater robot and the rate of change signal of the thruster control voltage, using a length L 1 The time domain window function of =400 intercepts the dynamic signal;
[0090] In the second step, wavelet decomposition is performed on the data in the time window, and the wavelet approximate component s is extracted A (n);
[0091] In the third step, the modified Bayesian algorithm is used to approximate the wavelet component s obtained in the second step A (n) to perform operations, the corrected Bayesian step size is N 2 , the calculated result is d sA (n), the calculation process is shown in formula (1) to formula (6).
[0092] The fourth step is to corr...
PUM
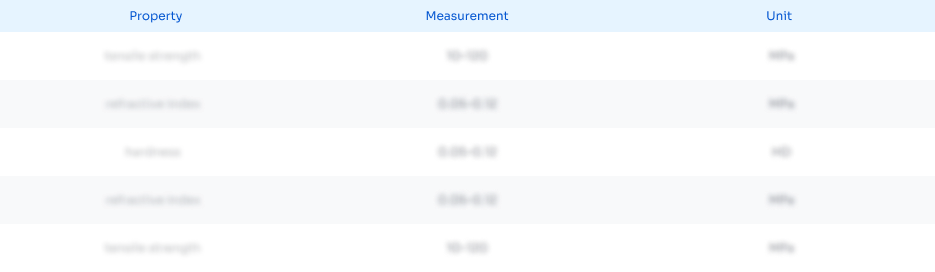
Abstract
Description
Claims
Application Information
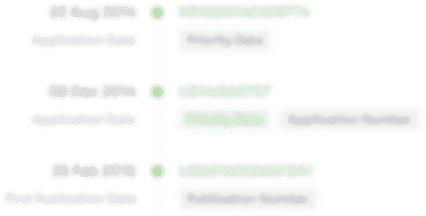
- R&D
- Intellectual Property
- Life Sciences
- Materials
- Tech Scout
- Unparalleled Data Quality
- Higher Quality Content
- 60% Fewer Hallucinations
Browse by: Latest US Patents, China's latest patents, Technical Efficacy Thesaurus, Application Domain, Technology Topic, Popular Technical Reports.
© 2025 PatSnap. All rights reserved.Legal|Privacy policy|Modern Slavery Act Transparency Statement|Sitemap|About US| Contact US: help@patsnap.com