BiGRU network drilling working condition identification method combined with attention mechanism
A technology of working condition recognition and attention, applied in character and pattern recognition, biological neural network model, manufacturing computing system, etc., can solve the problems of good model paper effect, small number of samples, and unknown
- Summary
- Abstract
- Description
- Claims
- Application Information
AI Technical Summary
Problems solved by technology
Method used
Image
Examples
Embodiment Construction
[0053] The preferred embodiments of the present invention will be described below with reference to the accompanying drawings. It should be understood that the preferred embodiments described herein are only used to illustrate and explain the present invention, but not to limit the present invention.
[0054] The BiGRU network drilling condition identification method combined with the attention mechanism provided by the present invention is specifically introduced as follows:
[0055] 1. Establish a time-series decision tree recognition model for drilling conditions combined with a semi-empirical formula combined with actual data, which is used to label drilling data.
[0056] In this example, the actual drilling data of six wells are used in total, and the data volume of each well is between 400,000 and 9,000,000. Data of this magnitude brings difficulties to the sample screening work, so the inventors It is proposed to use the typical criteria of drilling conditions to estab...
PUM
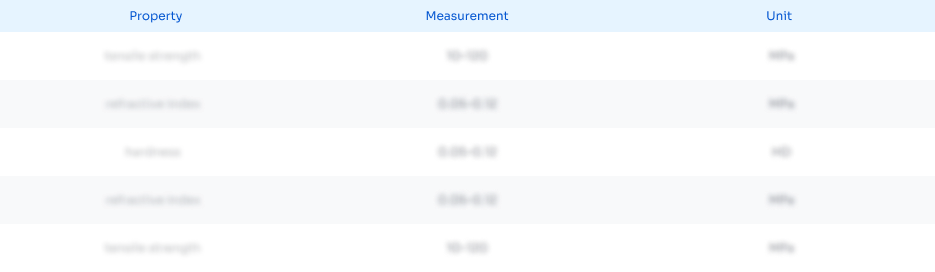
Abstract
Description
Claims
Application Information
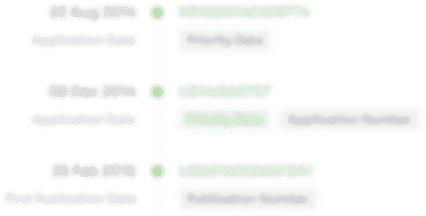
- R&D Engineer
- R&D Manager
- IP Professional
- Industry Leading Data Capabilities
- Powerful AI technology
- Patent DNA Extraction
Browse by: Latest US Patents, China's latest patents, Technical Efficacy Thesaurus, Application Domain, Technology Topic, Popular Technical Reports.
© 2024 PatSnap. All rights reserved.Legal|Privacy policy|Modern Slavery Act Transparency Statement|Sitemap|About US| Contact US: help@patsnap.com