Extreme multi-label learning method based on space-time network clustering reduction integration
A learning method and multi-label technology, applied in the field of multi-label text mining, can solve problems such as ignoring label sparsity, label-level model training, and poor learning scalability, so as to solve time and space consumption, improve representation ability, and improve general chemical effect
- Summary
- Abstract
- Description
- Claims
- Application Information
AI Technical Summary
Problems solved by technology
Method used
Image
Examples
Embodiment Construction
[0058] The technical solutions in the embodiments of the present invention will be clearly and completely described below with reference to the accompanying drawings in the embodiments of the present invention. Obviously, the described embodiments are only a part of the embodiments of the present invention, but not all of the embodiments. Based on the embodiments of the present invention, all other embodiments obtained by those of ordinary skill in the art without creative efforts shall fall within the protection scope of the present invention.
[0059] The present invention provides a technical solution: an extreme multi-label learning method based on space-time network clustering reduction integration, comprising the following steps:
[0060] S1: Space-time network attention ensemble representation;
[0061] S11: Acquisition of original extreme multi-label data; learning based on extreme multi-label data acquired in different actual application scenarios;
[0062] S12: Phra...
PUM
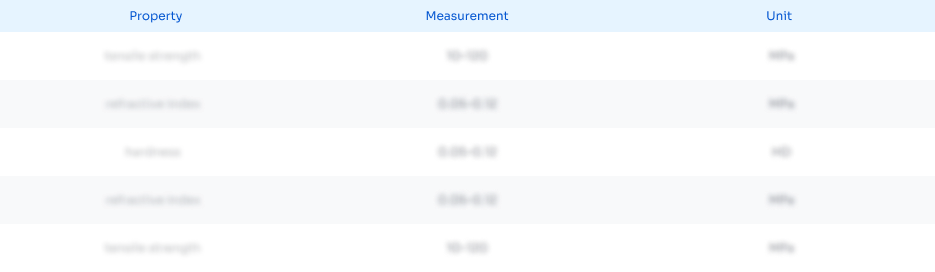
Abstract
Description
Claims
Application Information
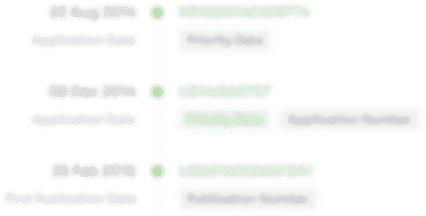
- Generate Ideas
- Intellectual Property
- Life Sciences
- Materials
- Tech Scout
- Unparalleled Data Quality
- Higher Quality Content
- 60% Fewer Hallucinations
Browse by: Latest US Patents, China's latest patents, Technical Efficacy Thesaurus, Application Domain, Technology Topic, Popular Technical Reports.
© 2025 PatSnap. All rights reserved.Legal|Privacy policy|Modern Slavery Act Transparency Statement|Sitemap|About US| Contact US: help@patsnap.com