Zero sample image recognition method and recognition device thereof, medium and computer terminal
A sample image and recognition method technology, applied in neural architecture, instruments, biological neural network models, etc., can solve the problems of incomplete category information of generated samples, inconsistent distribution of sample domains, etc., to speed up research and application, and increase class distinction , the effect of improving task performance
- Summary
- Abstract
- Description
- Claims
- Application Information
AI Technical Summary
Problems solved by technology
Method used
Image
Examples
Embodiment 1
[0112] The zero-sample image recognition method based on prototype domain alignment and cross-modal reconstruction of the present invention mainly has two stages: 1. a model training stage; 2. a zero-sample image recognition stage. Model training includes two parts: the training of the generative model and the training of the unseen class classifier. The first part is to obtain the trained feature generator. The second part first uses the trained feature generator and the semantic features of the unseen class to generate the unseen class. class visual features, and then train an unseen class classifier with these generated unseen class visual features. In the zero-sample image recognition stage, the unseen class image to be classified is sent to the trained unseen class classifier to identify the category to which the unseen class image belongs.
[0113] The zero-sample image recognition method can design a corresponding zero-sample image recognition device based on prototype ...
Embodiment 2
[0176] The zero-sample image recognition method in this embodiment is basically similar to that in Embodiment 1, and is used to recognize visual features of unseen classes. refer to Figure 4 , the method includes the following steps:
[0177] S1. Get the dataset.
[0178] S2. Extract visual features, semantic features and visual prototypes of visible class categories. Using the visual extractor and semantic extractor, the visual features and semantic features of the visible category images, and the unseen category semantic features are extracted. Design a prototype extractor to obtain visual prototypes for each visible class category.
[0179] S3. Use the visual features and semantic features of the visible category images and the visible category visual prototype to train the generative model to obtain a trained feature generator.
[0180] S4. Send the semantic features of the unseen category into the trained feature generator to obtain the generated visual features of t...
PUM
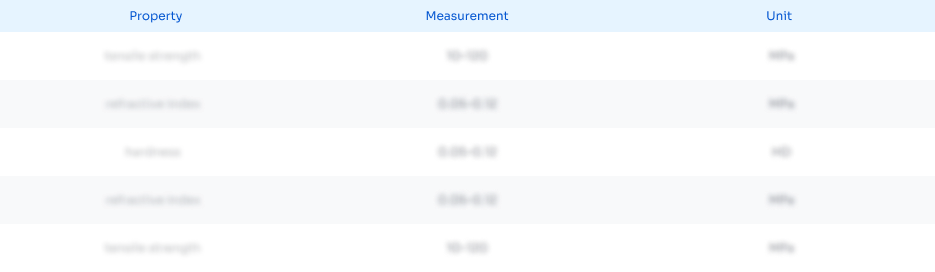
Abstract
Description
Claims
Application Information
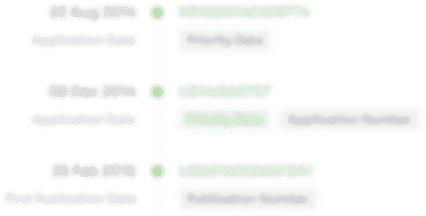
- R&D Engineer
- R&D Manager
- IP Professional
- Industry Leading Data Capabilities
- Powerful AI technology
- Patent DNA Extraction
Browse by: Latest US Patents, China's latest patents, Technical Efficacy Thesaurus, Application Domain, Technology Topic, Popular Technical Reports.
© 2024 PatSnap. All rights reserved.Legal|Privacy policy|Modern Slavery Act Transparency Statement|Sitemap|About US| Contact US: help@patsnap.com