Deep User Modeling by Behavior
- Summary
- Abstract
- Description
- Claims
- Application Information
AI Technical Summary
Benefits of technology
Problems solved by technology
Method used
Image
Examples
Example
DETAILED DESCRIPTION OF THE DRAWINGS
[0027]FIG. 1 illustrates a flow chart according to an exemplary embodiment of the present invention. As illustrated in FIG. 1, the process 100 includes obtaining user characteristics in step 101, transforming the user characteristics in step 102 using an attention based framework and producing a user behavior record in step 103. In step 104, the user behavior record is transformed using a modified sequence based LSTM network, which produces an observation matrix in step 105. LSTM networks are artificial recurrent neural network (RNN) architectures used in the field of deep learning. This enables deep learning of user characteristics represented by embedding. From the collected data as observation, we can estimate the modeling to minimize the loss between the target and the prediction, where the loss function is defined. In the data collection, we can take any data as a target, and leverage previous history as an input, and thus the framework is su...
PUM
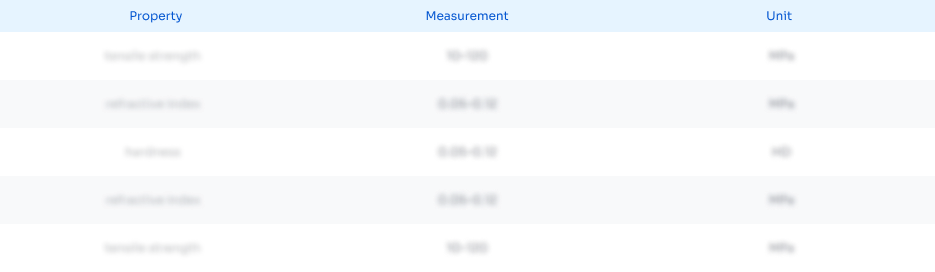
Abstract
Description
Claims
Application Information
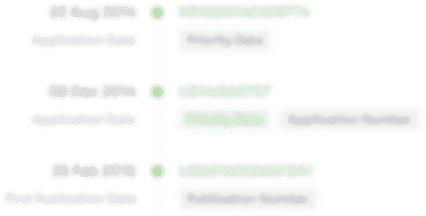
- R&D Engineer
- R&D Manager
- IP Professional
- Industry Leading Data Capabilities
- Powerful AI technology
- Patent DNA Extraction
Browse by: Latest US Patents, China's latest patents, Technical Efficacy Thesaurus, Application Domain, Technology Topic, Popular Technical Reports.
© 2024 PatSnap. All rights reserved.Legal|Privacy policy|Modern Slavery Act Transparency Statement|Sitemap|About US| Contact US: help@patsnap.com