Course monitoring method based on non-gauss component extraction and support vector description
A technology of support vector and process monitoring, applied in the direction of electrical testing/monitoring, etc., can solve the problems such as the separation result depends on the initial solution, the global optimality of the solution cannot be guaranteed, and the effective standard for selecting the number of pivot elements is lacking.
- Summary
- Abstract
- Description
- Claims
- Application Information
AI Technical Summary
Problems solved by technology
Method used
Image
Examples
Embodiment Construction
[0061] The present invention will be further described below in conjunction with the accompanying drawings.
[0062] refer to figure 1 , figure 2 as well as image 3 , a process monitoring method based on non-Gaussian component extraction and support vector data description, the specific implementation method is as follows:
[0063] (1) Offline modeling
[0064] Obtain a batch of measurement data of the industrial process, establish each model, and obtain the corresponding projection matrix. The specific process is as follows:
[0065] 1) Read the data of key variables during the normal operation of the production process as the training sample TX N×n , where N is the number of training samples and n is the number of variables;
[0066] 2) Preprocess the training sample TX so that the mean value of each variable is 0 and the variance is 1, and the input matrix X∈R is obtained N×n , the steps are:
[0067] (1) Calculate the mean: TX ‾ ...
PUM
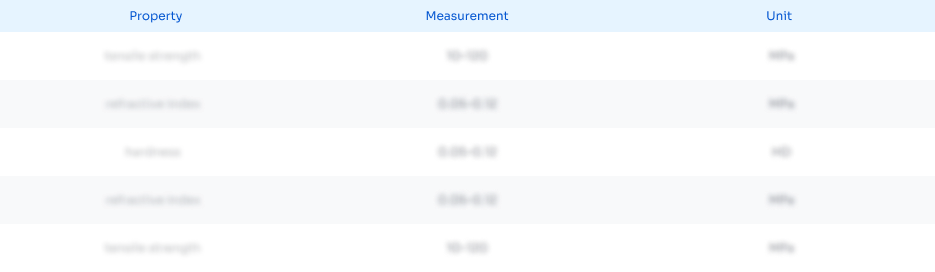
Abstract
Description
Claims
Application Information
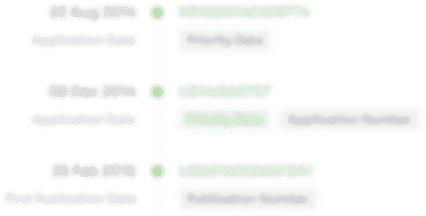
- R&D
- Intellectual Property
- Life Sciences
- Materials
- Tech Scout
- Unparalleled Data Quality
- Higher Quality Content
- 60% Fewer Hallucinations
Browse by: Latest US Patents, China's latest patents, Technical Efficacy Thesaurus, Application Domain, Technology Topic, Popular Technical Reports.
© 2025 PatSnap. All rights reserved.Legal|Privacy policy|Modern Slavery Act Transparency Statement|Sitemap|About US| Contact US: help@patsnap.com