N-gram-based semantic mining method for increment of topic model
A topic model and semantic mining technology, applied in special data processing applications, instruments, electrical digital data processing, etc., can solve the problem of long training time of topic models
- Summary
- Abstract
- Description
- Claims
- Application Information
AI Technical Summary
Problems solved by technology
Method used
Image
Examples
Embodiment Construction
[0041] Embodiments of the present invention will be described in detail below with reference to the accompanying drawings.
[0042] Studies have shown that the semantic expression ability of N-gram (N-grammatical elements) is stronger than that of simple words (Unigram, that is, unary grammatical elements), and the word space composed of N-grams (N-grammatical elements) can effectively improve text mining. Effects, such as: text clustering, text classification, text retrieval. However, directly introducing N-grams (N-gram elements) into the topic model will greatly increase the complexity of model training, making it difficult for the topic model to be directly applied to text mining tasks that require high resource requirements. At the same time, the research also shows that the prior probability value of each random variable in the topic model can control the topic distribution in the final training model, so the prior knowledge of historical data can be recorded by using th...
PUM
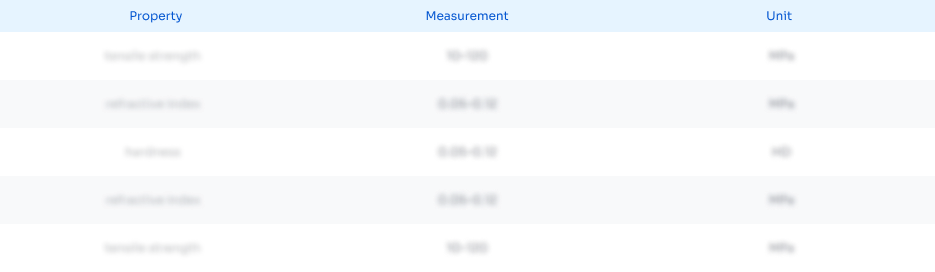
Abstract
Description
Claims
Application Information
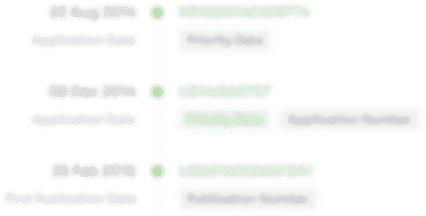
- R&D Engineer
- R&D Manager
- IP Professional
- Industry Leading Data Capabilities
- Powerful AI technology
- Patent DNA Extraction
Browse by: Latest US Patents, China's latest patents, Technical Efficacy Thesaurus, Application Domain, Technology Topic.
© 2024 PatSnap. All rights reserved.Legal|Privacy policy|Modern Slavery Act Transparency Statement|Sitemap