Building method of two-level fitting quantitative structure-activity relationship (QSAR) model for forecasting compound activity
A technology for building a method and fitting a model, applied in the field of biomedical information, it can solve the problems of model performance degradation, modeling failure, non-convergence, etc., to achieve the effect of fewer independent variables, prevention of modeling failure, and easy interpretation.
- Summary
- Abstract
- Description
- Claims
- Application Information
AI Technical Summary
Problems solved by technology
Method used
Image
Examples
Embodiment 1
[0039] like figure 1 As shown, the linear regression-neural network of the present embodiment fits the QSAR model in two stages before and after, and its construction steps are as follows:
[0040] 1) Finishing of biological activity
[0041] In order to ensure the statistical effect, 35 pyrazole compounds with p38 kinase inhibitory rate were taken as the training set S 1 , convert its inhibition rate α into logarithmic form: Y 1 =LgBio=-lg(α -1 -1). Y 1 =LgBio is the dependent variable used in subsequent modeling. Sybyl analysis software was used to test the two-dimensional structure of the compound, and the three-dimensional structure of the compound that passed the test was generated.
[0042] 2) Construction of pre-fitting model
[0043] The training set compound S 1 Import the molecular table S1.tbl of Sybyl software, in the Topomer CoMFA module, for the training set S 1 The substituents of the compounds are divided. On the one hand, the division of substituents m...
Embodiment 2
[0047] This embodiment is to measure the goodness of fit, and compare the M 1 -M 2 Two-level model with M 1 The goodness of fit of the single-level model, the specific steps are as follows:
[0048] 1) Variable naming
[0049] will model M 1 For the training set S 1 The calculated activity of the compound is named Y 2 .
[0050] will model M 2 For the training set S 1 The calculated activity of the compound is named Y 3 .
[0051] 2) Export spreadsheet file
[0052] The Sybyl molecule forms S 1 The two columns LgBio and Pre_LgBio in .tbl are exported as S 1 _M 1 .csv file, then converted to S 1 _M 1 .xls file. The above LgBio is Y 1 , Pre_LgBio is Y 2 .
[0053] Using the same method, export M2 from the SPSS Clementine software to the training set compound S 1 The computational activity of , saved as S 1 _M 2 .xls file; where, S 1 _M 2 The .xls file contains the variable Y 1 and Y 3 .
[0054] 3) Calculate the square of the correlation coefficient an...
Embodiment 3
[0059] This embodiment is to measure the predictive performance, compare the above-mentioned embodiment 1 built M 1 -M 2 Two-level model with M 1 The prediction performance of the single-level model, the specific steps are as follows:
[0060] 1) Collation of p38 kinase inhibitory activity
[0061] Take 35 non-training sets S 1 Pyrazole compounds of elements form a test set S 2 , whose p38 kinase inhibitory activity is denoted as Y 4 . The test set S 2 The 35 pyrazole compounds are made into Sybyl molecular form S 2 .tbl, Y 4 Specified as dependent variable (indicated as LgBio in the S2.tbl molecule sheet).
[0062] 2) Determination of the predictive performance of the single-level model M1
[0063] In the TopomerCoMFA module of Sybyl software, the predicted molecular form S 2 .p38 kinase inhibitory activity of tbl, the result is marked as Y 5 (at S 2 Indicated as Pre_LgBio in the .tbl molecule form). During the prediction process, the local physiological effects...
PUM
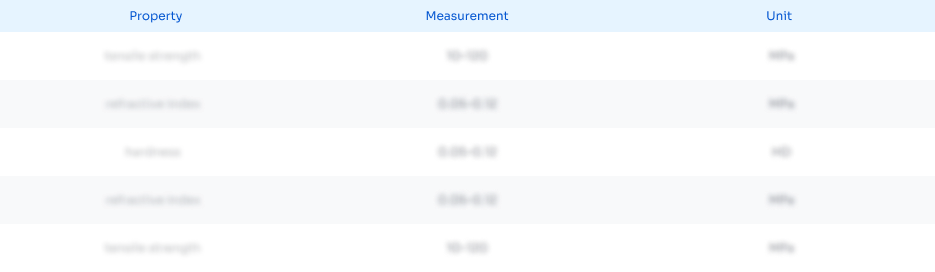
Abstract
Description
Claims
Application Information
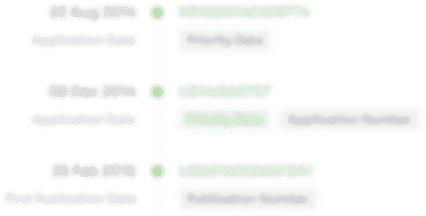
- R&D Engineer
- R&D Manager
- IP Professional
- Industry Leading Data Capabilities
- Powerful AI technology
- Patent DNA Extraction
Browse by: Latest US Patents, China's latest patents, Technical Efficacy Thesaurus, Application Domain, Technology Topic, Popular Technical Reports.
© 2024 PatSnap. All rights reserved.Legal|Privacy policy|Modern Slavery Act Transparency Statement|Sitemap|About US| Contact US: help@patsnap.com