Probability hypothesis density multi-target tracking method based on variational Bayesian approximation technology
A technology of probability assumption density and variational Bayesian technology, applied in the field of variable number target tracking with unknown measurement noise, to reduce computational complexity and improve operating efficiency
- Summary
- Abstract
- Description
- Claims
- Application Information
AI Technical Summary
Problems solved by technology
Method used
Image
Examples
Embodiment Construction
[0029] 1. Introduction to basic theory
[0030] 1. Variational Bayesian approximation technique
[0031] Suppose the state equation and measurement equation of a single target are expressed as:
[0032] x k+1 Fx k +Gw k 1)
[0033] the y k =h(x k )+v k 2) Among them, represents the state vector of the target at time k, F is a one-step transition matrix, the function h( ) represents the observation model, w k and v k Denote the state noise and measurement noise, respectively, and the corresponding covariances are denoted as Q k and R k . In real tracking scenarios, R k Usually unknown and changing, need to be estimated in due course.
[0034] Assuming that the target dynamic model is independent of the measurement noise covariance, the target state x k and the measurement noise covariance R k The joint posterior probability distribution of can be expressed as:
[0035] p(x k , R k |y 1:k-1 )=∫p(x k |x k-1 )p(R k |R k-1 )p(x k-1 , R k-1 |y 1:k-1 ...
PUM
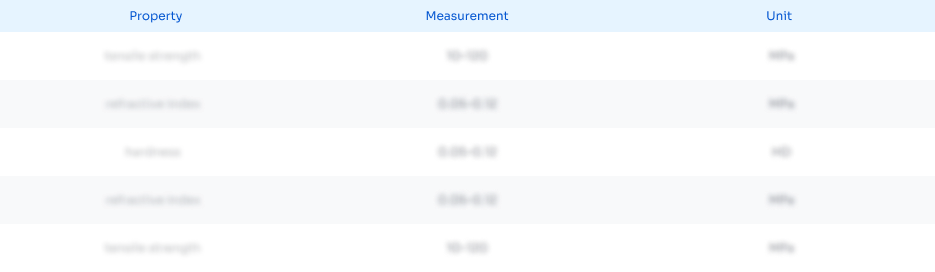
Abstract
Description
Claims
Application Information
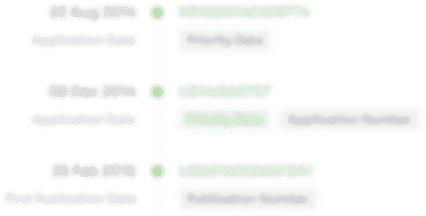
- R&D Engineer
- R&D Manager
- IP Professional
- Industry Leading Data Capabilities
- Powerful AI technology
- Patent DNA Extraction
Browse by: Latest US Patents, China's latest patents, Technical Efficacy Thesaurus, Application Domain, Technology Topic, Popular Technical Reports.
© 2024 PatSnap. All rights reserved.Legal|Privacy policy|Modern Slavery Act Transparency Statement|Sitemap|About US| Contact US: help@patsnap.com