Method and device for data identification based on multitask deep neural network
A deep neural network, data recognition technology, applied in the field of pattern recognition and machine learning, can solve the problem of not considering the correlation of labels and reducing the expression ability of the model
- Summary
- Abstract
- Description
- Claims
- Application Information
AI Technical Summary
Problems solved by technology
Method used
Image
Examples
Embodiment Construction
[0018] In order to make the object, technical solution and advantages of the present invention clearer, the present invention will be further described in detail below in conjunction with specific embodiments and with reference to the accompanying drawings.
[0019] The invention proposes a data recognition method based on a multi-task deep neural network, which can be widely applied to multi-category labeling problems.
[0020] figure 1 A flow chart of the steps of the data recognition method based on the multi-task deep neural network proposed by the present invention is shown. Such as figure 1 As shown, the method includes:
[0021] Step 1, establish a multi-task deep neural network, set the number of layers and the number of nodes of the network; wherein the multi-task deep neural network is a multi-layer network structure, and the input layer can be the pixel points of the corresponding image, and all input images are required to remain the same The size of the input l...
PUM
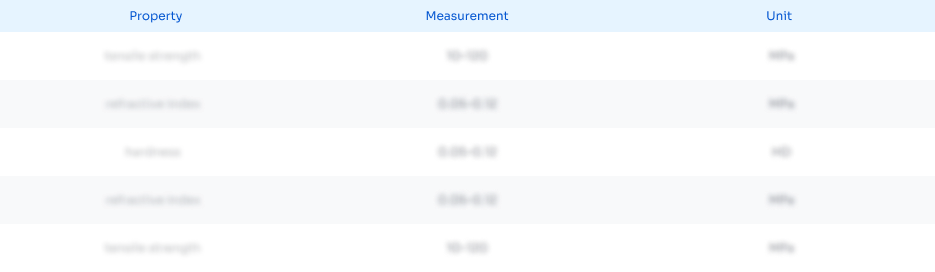
Abstract
Description
Claims
Application Information
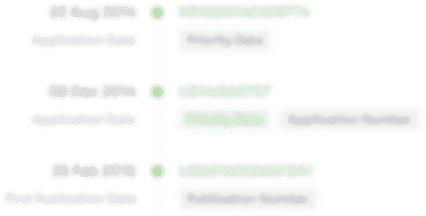
- R&D Engineer
- R&D Manager
- IP Professional
- Industry Leading Data Capabilities
- Powerful AI technology
- Patent DNA Extraction
Browse by: Latest US Patents, China's latest patents, Technical Efficacy Thesaurus, Application Domain, Technology Topic.
© 2024 PatSnap. All rights reserved.Legal|Privacy policy|Modern Slavery Act Transparency Statement|Sitemap