Office building load prediction method based on particle swarm neural network
A neural network and load forecasting technology, applied in biological neural network models, forecasting, data processing applications, etc., can solve the problems that are not easy to implement, the load forecasting accuracy cannot reach high-precision load forecasting algorithms, and are complex, and achieve load forecasting. The effect of high precision, easy realization and high precision load forecasting
- Summary
- Abstract
- Description
- Claims
- Application Information
AI Technical Summary
Problems solved by technology
Method used
Image
Examples
Embodiment Construction
[0023] The present invention will be further described below in conjunction with the accompanying drawings. The following examples are only used to illustrate the technical solution of the present invention more clearly, but not to limit the protection scope of the present invention.
[0024] The scheme flowchart of the present invention is as figure 1 As shown, it mainly includes the following seven steps, and the specific implementation is as follows:
[0025] Step 1: Determine the input feature variables and output target vector
[0026] This embodiment is a certain office building in Beijing. Generally speaking, the building load is caused by the external and internal disturbances of the building. The main reasons for the fluctuation of the building load are the changes of outdoor meteorological parameters and indoor personnel and the start and stop of equipment. For office buildings, personnel changes are relatively regular, and building loads often show cyclical chang...
PUM
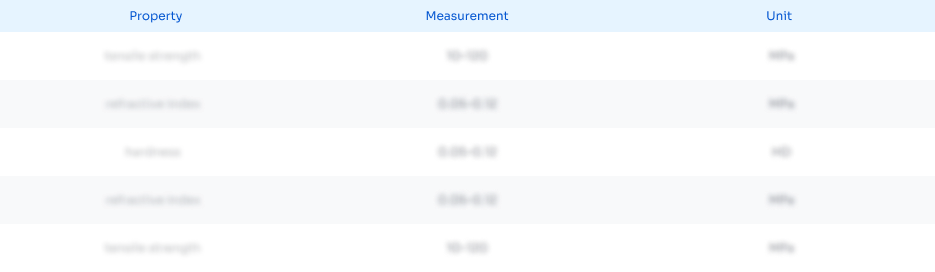
Abstract
Description
Claims
Application Information
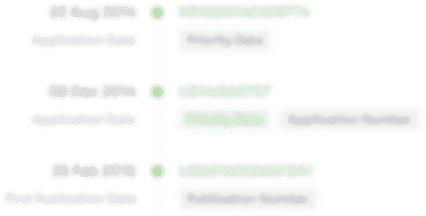
- R&D Engineer
- R&D Manager
- IP Professional
- Industry Leading Data Capabilities
- Powerful AI technology
- Patent DNA Extraction
Browse by: Latest US Patents, China's latest patents, Technical Efficacy Thesaurus, Application Domain, Technology Topic, Popular Technical Reports.
© 2024 PatSnap. All rights reserved.Legal|Privacy policy|Modern Slavery Act Transparency Statement|Sitemap|About US| Contact US: help@patsnap.com