Data center station multivariate load prediction method based on hybrid model prediction
A load forecasting and hybrid model technology, applied in forecasting, data processing applications, character and pattern recognition, etc., can solve the problems of dependence on data characteristics, long adjustment time, local minimum output layer of neural network, etc., and achieve good nonlinear fitting. capacity, high-precision load forecasting, and the effect of efficient data mining
- Summary
- Abstract
- Description
- Claims
- Application Information
AI Technical Summary
Problems solved by technology
Method used
Image
Examples
Embodiment Construction
[0094] The specific implementation manners of the present invention will be further described in detail below in conjunction with the accompanying drawings and embodiments. The following examples are used to illustrate the present invention, but are not intended to limit the scope of the present invention.
[0095] A multivariate load forecasting method for data center stations based on hybrid model forecasting, such as figure 1 shown, including the following steps:
[0096] Step 1: Data collection and data preprocessing; obtain the historical data of the data center station within the preset time and build a training set, and preprocess the data, where the historical data includes cooling load, heating load, electrical load, light intensity, wind speed, Humidity, barometric pressure and date.
[0097] Step 1.1: Obtain the historical data of the data center station within the preset time, and use the clustering algorithm K-means method to divide the load of the data center s...
PUM
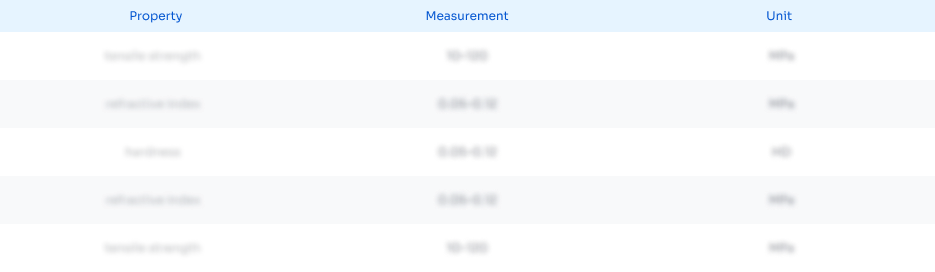
Abstract
Description
Claims
Application Information
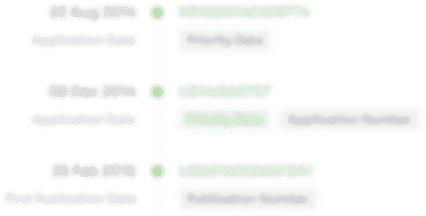
- R&D
- Intellectual Property
- Life Sciences
- Materials
- Tech Scout
- Unparalleled Data Quality
- Higher Quality Content
- 60% Fewer Hallucinations
Browse by: Latest US Patents, China's latest patents, Technical Efficacy Thesaurus, Application Domain, Technology Topic, Popular Technical Reports.
© 2025 PatSnap. All rights reserved.Legal|Privacy policy|Modern Slavery Act Transparency Statement|Sitemap|About US| Contact US: help@patsnap.com