IES incomplete data load prediction method and system based on C-GAN transfer learning
A C-GAN, load forecasting technology, applied in neural learning methods, forecasting, data processing applications, etc., can solve problems such as insufficient speed, inability to apply complex systems, and failure to consider incomplete data conditions
- Summary
- Abstract
- Description
- Claims
- Application Information
AI Technical Summary
Problems solved by technology
Method used
Image
Examples
Embodiment Construction
[0093] The invention will be further described below in conjunction with the accompanying drawings and specific implementation examples.
[0094] Such as figure 1 As shown, the IES incomplete data load prediction method based on C-GAN transfer learning includes the following steps:
[0095] Step 1: Collect the original sample data set, the original sample data set includes the historical sample data set of the integrated energy system and the historical sample data set of the actual load characteristic data, the historical sample data set of the integrated energy system includes four sample data The data sets are the temperature sample data set, the humidity sample data set, the date sample data set and the economic sample data set of the integrated energy system respectively. The historical sample data set of the actual load characteristic data includes three sample data sets, which are respectively Sample Dataset, Air Load Sample Dataset, and Heat Load Sample Dataset;
[0...
PUM
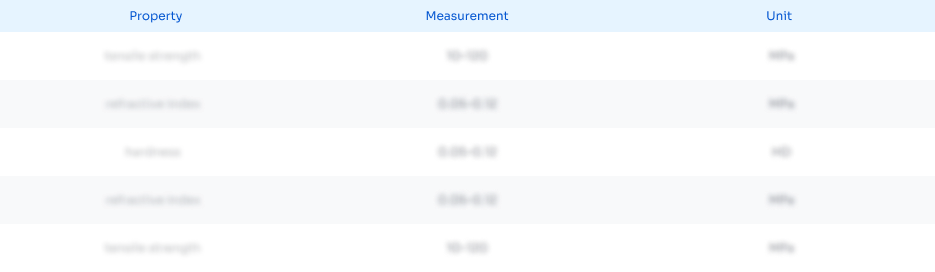
Abstract
Description
Claims
Application Information
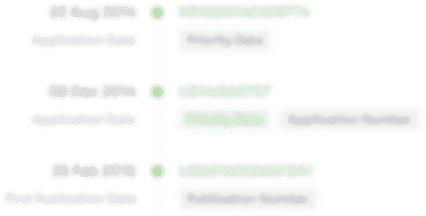
- R&D Engineer
- R&D Manager
- IP Professional
- Industry Leading Data Capabilities
- Powerful AI technology
- Patent DNA Extraction
Browse by: Latest US Patents, China's latest patents, Technical Efficacy Thesaurus, Application Domain, Technology Topic.
© 2024 PatSnap. All rights reserved.Legal|Privacy policy|Modern Slavery Act Transparency Statement|Sitemap