Manifold-based linear regression learning method
A linear regression and learning method technology, applied in the field of manifold-based linear regression learning, can solve problems such as multicollinearity, and achieve the effect of effective identification, simple and feasible practicability
- Summary
- Abstract
- Description
- Claims
- Application Information
AI Technical Summary
Benefits of technology
Problems solved by technology
Method used
Image
Examples
Embodiment Construction
[0018] The following describes in detail a manifold-based linear regression learning method of the present invention with reference to embodiments and drawings.
[0019] The present invention proposes a manifold-based linear regression classification model. There are two main sources of ideas. One is that the linear regression model can be used to map samples of the same class to a linear subspace. This feature can be used to obtain better The classification effect, the second is that manifold learning is an important non-linear feature extraction classification technology, which can be used to map high-dimensional features to low-dimensional manifold spaces that can reveal the internal structure between data sets. Many image data sets present a nonlinear structure in the original high-dimensional space. Manifold learning can maintain the nonlinear structure in the high-dimensional space through feature mapping, and has been widely used in image analysis and pattern recognition.
...
PUM
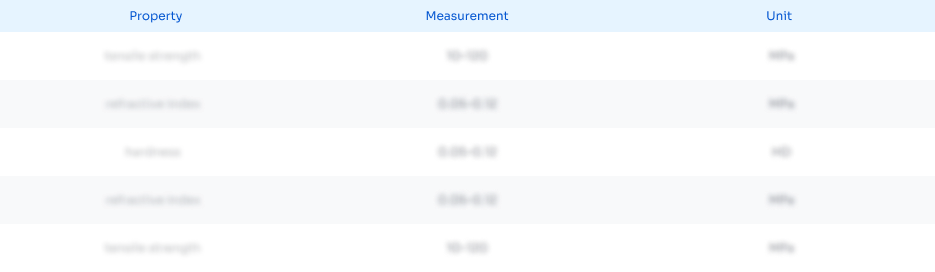
Abstract
Description
Claims
Application Information
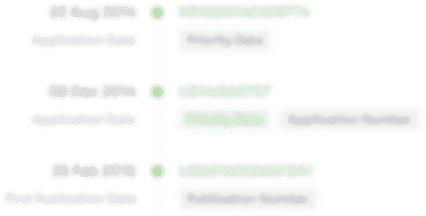
- R&D Engineer
- R&D Manager
- IP Professional
- Industry Leading Data Capabilities
- Powerful AI technology
- Patent DNA Extraction
Browse by: Latest US Patents, China's latest patents, Technical Efficacy Thesaurus, Application Domain, Technology Topic.
© 2024 PatSnap. All rights reserved.Legal|Privacy policy|Modern Slavery Act Transparency Statement|Sitemap