Method for predicting fluctuating wind speed based on optimization-based multiple kernel LSSVM (Least Square Support Vector Machine)
A technology of pulsating wind speed and prediction method, which is applied in special data processing applications, instruments, electrical digital data processing, etc., and can solve the problems of increasing cost and time-consuming
- Summary
- Abstract
- Description
- Claims
- Application Information
AI Technical Summary
Problems solved by technology
Method used
Image
Examples
Embodiment Construction
[0027] The idea of the present invention is as follows: a single kernel function is often difficult to satisfy good learning ability and strong generalization ability at the same time, and the combination of different kernel functions can make the multi-core kernel function include the advantages of different single-core kernel functions. By optimizing the weights of each core, the most suitable parameters can be obtained to make multi-core learning more stable. According to Mercer's theorem, any kernel function k(x i ,x j ) Gram matrix K is symmetric and positive semi-definite, and satisfies a certain number of enclosing properties, which allow complex kernels to be created from simple kernels. Therefore, the linear combination of RBF_kernel, Poly_kernel, and Lin_kernel kernel functions constructs a new kernel function, so that the prediction model has both good learning ability (small training error) and strong generalization ability (small test error). , while improving...
PUM
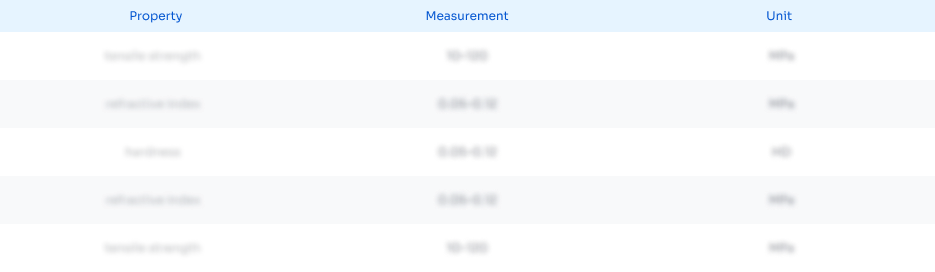
Abstract
Description
Claims
Application Information
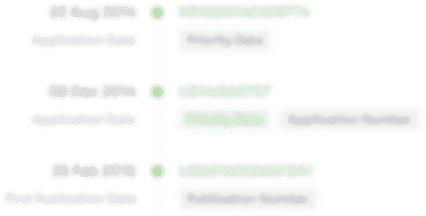
- R&D Engineer
- R&D Manager
- IP Professional
- Industry Leading Data Capabilities
- Powerful AI technology
- Patent DNA Extraction
Browse by: Latest US Patents, China's latest patents, Technical Efficacy Thesaurus, Application Domain, Technology Topic, Popular Technical Reports.
© 2024 PatSnap. All rights reserved.Legal|Privacy policy|Modern Slavery Act Transparency Statement|Sitemap|About US| Contact US: help@patsnap.com