Microgrid Load Forecasting System and Method Based on Online Power Purchase and Dynamic Correlation Factors
A technology of dynamic association and load forecasting, applied in forecasting, neural learning methods, biological neural network models, etc., can solve the problems of reduced forecasting accuracy, no consideration of users' future electricity consumption information, and slow convergence speed
- Summary
- Abstract
- Description
- Claims
- Application Information
AI Technical Summary
Problems solved by technology
Method used
Image
Examples
Embodiment Construction
[0068] The specific embodiments of the present invention will be described in detail below in conjunction with the accompanying drawings.
[0069] Microgrid load forecasting system based on online power purchase and dynamic correlation factors, such as figure 1 As shown in Fig. 1, it includes an online power purchase module, a load characteristic analysis module, a short-term load forecast module and a forecast result output module.
[0070] In this embodiment, the online power purchase module is to establish an interaction mechanism between power users and the microgrid, which is used to communicate with power users through the microgrid, establish basic information and power information of the users, and based on the load information obtained through the microgrid and load impact factor information, make statistics on various loads, obtain historical load data, and provide users with preliminary power orders at the same time, and provide users with preliminary power orders a...
PUM
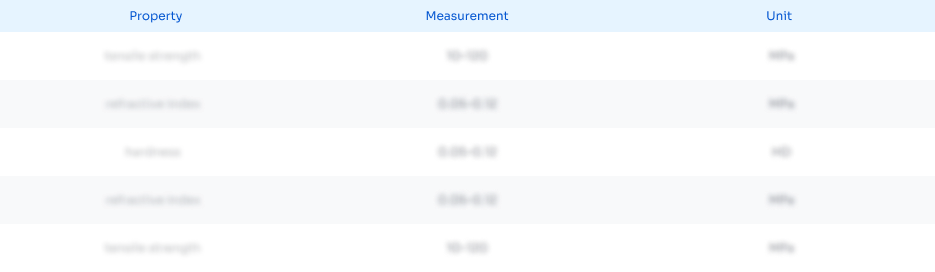
Abstract
Description
Claims
Application Information
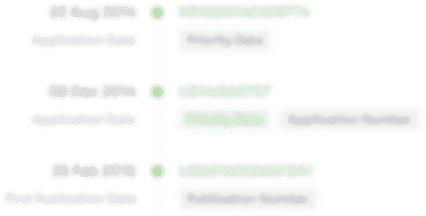
- R&D Engineer
- R&D Manager
- IP Professional
- Industry Leading Data Capabilities
- Powerful AI technology
- Patent DNA Extraction
Browse by: Latest US Patents, China's latest patents, Technical Efficacy Thesaurus, Application Domain, Technology Topic, Popular Technical Reports.
© 2024 PatSnap. All rights reserved.Legal|Privacy policy|Modern Slavery Act Transparency Statement|Sitemap|About US| Contact US: help@patsnap.com