Multi-source Image Change Detection Method for Cluster-Guided Deep Neural Network Classification
A technology of deep neural network and change detection, which is applied in the field of multi-source image change detection of cluster-guided deep neural network classification, can solve the problems of dimensionality reduction, difficulty in adapting to production requirements, difficulty in meeting precision, etc., and achieve accuracy High, excellent feature learning ability, and the effect of solving classification problems
- Summary
- Abstract
- Description
- Claims
- Application Information
AI Technical Summary
Problems solved by technology
Method used
Image
Examples
Embodiment 1
[0030] The present invention is a multi-source image change detection method for clustering-guided deep neural network classification. In the present invention, the multi-source image to be processed includes an optical image and a TM image. Grayscale matrix of optical images in images acquired by different sensors. see figure 1 , image change detection includes the following steps:
[0031] (1) Input the optical image to be tested, hereinafter referred to as the optical image: convert the optical image whose data type is three-dimensional into a grayscale matrix whose data type is two-dimensional, and input the grayscale matrix of the optical image.
[0032] (2) Segmentation of optical images: Fuzzy C-means clustering method is used to perform fuzzy clustering on the grayscale matrix of the optical image to be detected to obtain the grayscale matrix after clustering and segmentation of optical images. The fuzzy C-means clustering method is referred to as FCM .
[0033] (3)...
Embodiment 2
[0046] The multi-source image change detection method that clustering guides deep neural network classification is the same as embodiment 1, wherein the process of the training stacked autoencoder SAE described in step (6) includes:
[0047] (6a) The stacked autoencoder is composed of two layers of sparse autoencoders, and the activation value of the hidden layer nodes of the first layer of sparse autoencoder is used as the input of the second layer of sparse autoencoder.
[0048] (6b) Set the input layer of the first layer of sparse autoencoder to 9 nodes, the hidden layer to 49 nodes, the input layer of the second layer of sparse autoencoder and the hidden layer of the first layer of sparse autoencoder The nodes are the same, set to 49 nodes, and the hidden layer of the second-layer sparse autoencoder is set to 10 nodes.
[0049] (6c) Initialize the weights on the stacked autoencoder SAE with random numbers on the interval [0, 1].
[0050] (6d) Input the selected training s...
Embodiment 3
[0056] The multi-source image change detection method of clustering-guided deep neural network classification is the same as embodiment 1-2, wherein the specific steps of carrying out block sampling to image data described in step (4) include:
[0057] 4.1: If image X 0 The dimension is p×q. In this example, 3×3 is taken as an example to describe in detail. An all-zero matrix X with a dimension of (p+2)×(q+2) is initialized.
[0058] 4.2: Put the image matrix X 0 The value of each element in is assigned to all elements in the rectangular range from the 2nd row to the p+1th row, the 2nd column to the q+1th column in the all-zero matrix X;
[0059] 4.3: Assign all elements of row 3 in the all-zero matrix X to all elements of row 1, and assign all elements of row p to all elements of row p+2.
[0060] 4.4: Assign all the elements in the third column of X to all the elements in the first column, and assign all the elements in the qth column to all the elements in the q+2th colum...
PUM
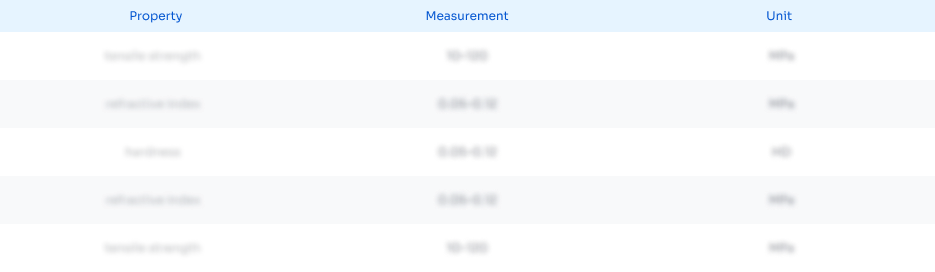
Abstract
Description
Claims
Application Information
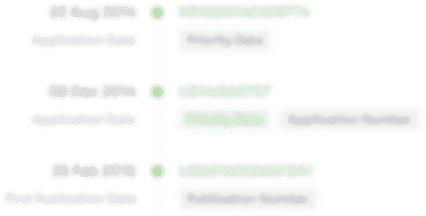
- R&D Engineer
- R&D Manager
- IP Professional
- Industry Leading Data Capabilities
- Powerful AI technology
- Patent DNA Extraction
Browse by: Latest US Patents, China's latest patents, Technical Efficacy Thesaurus, Application Domain, Technology Topic, Popular Technical Reports.
© 2024 PatSnap. All rights reserved.Legal|Privacy policy|Modern Slavery Act Transparency Statement|Sitemap|About US| Contact US: help@patsnap.com