Possibility fuzzy c mean clustering algorithm based on multiple kernels
A possibility, multi-core technology, applied in computing, computer components, character and pattern recognition, etc.
- Summary
- Abstract
- Description
- Claims
- Application Information
AI Technical Summary
Problems solved by technology
Method used
Image
Examples
Embodiment Construction
[0040] In this embodiment, the variant data set and the glass identification data set are used for experimental description. The variant data set has a total of 160 data points, divided into 9 attributes, and a total of eight categories. The glass identification data set has a total of 214 points, divided into 9 There are 6 categories in total.
[0041] A multi-kernel possibility fuzzy clustering algorithm is carried out according to the following steps:
[0042] Step 1. Let X={x 1 ,x 2 ,...,x j ,...,x n} represents a given sample set, x j Indicates the j-th sample; 1≤j≤n, n is the number of samples; optimally divide the sample set X so that the objective function value J shown in formula (1) is the smallest, and the clustering obtained by the smallest objective function value The center is the best, and the effect of dividing the data is also the best. The classification effect is shown in Table 1 and Table 2:
[0043] Table 1 Clustering accuracy results experiment
[...
PUM
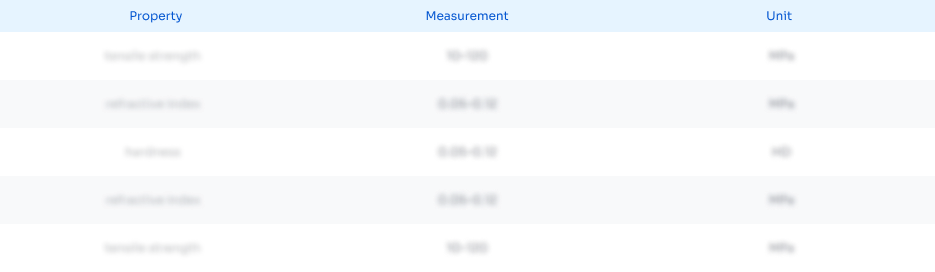
Abstract
Description
Claims
Application Information
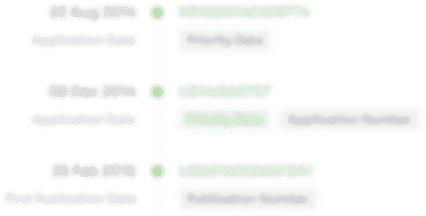
- R&D
- Intellectual Property
- Life Sciences
- Materials
- Tech Scout
- Unparalleled Data Quality
- Higher Quality Content
- 60% Fewer Hallucinations
Browse by: Latest US Patents, China's latest patents, Technical Efficacy Thesaurus, Application Domain, Technology Topic, Popular Technical Reports.
© 2025 PatSnap. All rights reserved.Legal|Privacy policy|Modern Slavery Act Transparency Statement|Sitemap|About US| Contact US: help@patsnap.com