A software defect prediction method and prediction system
A software defect prediction and defect-free technology, applied in software testing/debugging, neural learning methods, computer components, etc., can solve the problem of not considering the local relationship of data samples, not taking into account the sample category information, and not being able to handle it well sample data etc.
- Summary
- Abstract
- Description
- Claims
- Application Information
AI Technical Summary
Problems solved by technology
Method used
Image
Examples
Embodiment Construction
[0073] The technical solutions of the present invention will be described in detail below in conjunction with the accompanying drawings.
[0074] Such as figure 1 Shown, the present invention comprises the following steps:
[0075] 1. Use the training sample set X to construct an adjacency graph: samples in X are divided into defective samples, non-defective samples, and unlabeled samples, and three adjacency graphs a, b, and c need to be constructed.
[0076] For the adjacency graph a, all the samples in the sample set are used as the nodes of the adjacency graph, if there are two nodes belonging to the same sample and the neighbors are connected, a connection edge is established;
[0077] For the adjacency graph b, all the samples in the sample set are used as the nodes of the adjacency graph. If there are two nodes belonging to heterogeneous samples and the neighbors are adjacent, a connection edge is established;
[0078] For the adjacency graph c, all the samples in the...
PUM
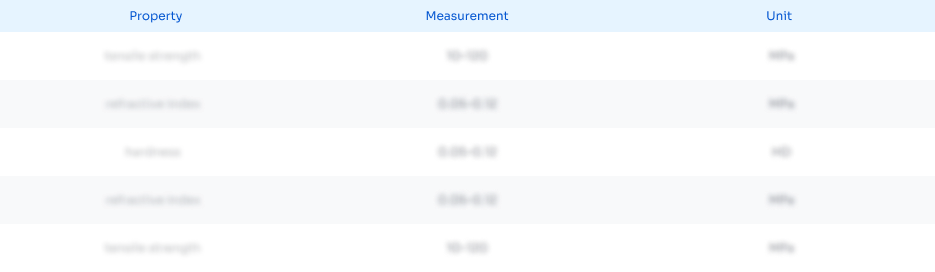
Abstract
Description
Claims
Application Information
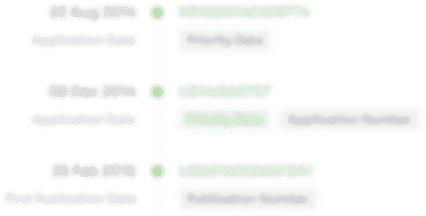
- R&D Engineer
- R&D Manager
- IP Professional
- Industry Leading Data Capabilities
- Powerful AI technology
- Patent DNA Extraction
Browse by: Latest US Patents, China's latest patents, Technical Efficacy Thesaurus, Application Domain, Technology Topic, Popular Technical Reports.
© 2024 PatSnap. All rights reserved.Legal|Privacy policy|Modern Slavery Act Transparency Statement|Sitemap|About US| Contact US: help@patsnap.com