Rotating-machinery life-stage identification method based on deep self-encoding learning network of noise enhanced samples
A technology for learning networks and rotating machinery, applied in neural learning methods, biological neural network models, special data processing applications, etc.
- Summary
- Abstract
- Description
- Claims
- Application Information
AI Technical Summary
Problems solved by technology
Method used
Image
Examples
Embodiment
[0116] In this embodiment, the validity of the present invention is verified by the following steps:
[0117] The first step: collect data, add noise sample enhancement. Obtained a certain type of rolling bearing full-life experimental sample, the bearing was continuously operated for 90 days under the conditions of a rotating speed of 3000rpm and a load of 3kg, and vibration signals were collected every 4 hours, and two groups of signals were collected in sequence each time, and the sampling frequency was 51.2kHz. The sample length is 100k. After obtaining the amplitude spectrum of the first group of vibration signals collected each time, the graphs are drawn sequentially according to the sampling time to obtain the spectrogram as shown in figure 2 shown. Observing the figure, with the increase of the bearing running time (number of running circles), the bearing gradually wears out, and the spectrum energy and spectrum structure of the vibration signal have changed. The ge...
PUM
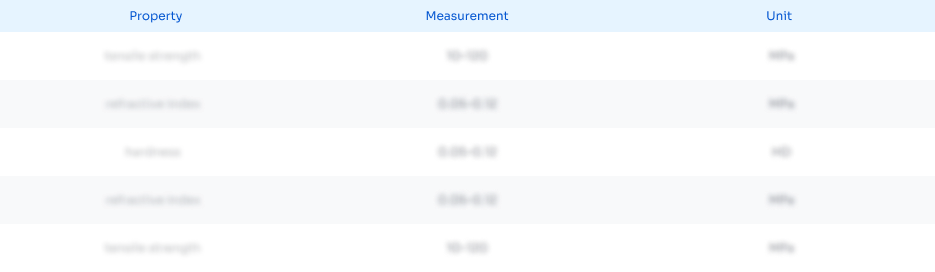
Abstract
Description
Claims
Application Information
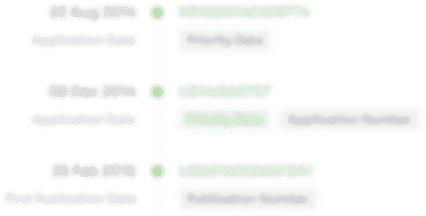
- R&D
- Intellectual Property
- Life Sciences
- Materials
- Tech Scout
- Unparalleled Data Quality
- Higher Quality Content
- 60% Fewer Hallucinations
Browse by: Latest US Patents, China's latest patents, Technical Efficacy Thesaurus, Application Domain, Technology Topic, Popular Technical Reports.
© 2025 PatSnap. All rights reserved.Legal|Privacy policy|Modern Slavery Act Transparency Statement|Sitemap|About US| Contact US: help@patsnap.com