Method for establishing oil deposit development effect prediction model
A technology for reservoir development and prediction model, applied in the field of oil and gas exploration and development, which can solve the problems of insufficient data volume, short time, and inability to truly and effectively describe the core laws of training samples.
- Summary
- Abstract
- Description
- Claims
- Application Information
AI Technical Summary
Problems solved by technology
Method used
Image
Examples
Embodiment 1
[0045] figure 1 A flow chart of building a reservoir development effect prediction model provided by this embodiment is shown.
[0046] Such as figure 1 As shown, the method provided in this embodiment first uses known reservoir development data to perform K-fold cross-training on the initial neural network in step S101 to obtain K sets of network parameters corresponding to K sets of verification data.
[0047] specifically, figure 2 A flow chart of K-fold cross-training of the initial neural network by using known reservoir development data in step S101 in this embodiment is shown.
[0048] From figure 2It can be seen from the figure that when K-fold cross-training is performed on the initial neural network, the known reservoir development data is firstly divided into K groups in step S201, thereby obtaining K groups of data. In this embodiment, the natural number K is preferably configured as 4. Of course, in other embodiments of the present invention, according to a...
Embodiment 2
[0065] image 3 A flow chart of building a reservoir development effect prediction model provided by this embodiment is shown.
[0066] Such as image 3 As shown, the method provided in this embodiment first divides the known reservoir development data into K groups in step S301, thereby obtaining K groups of data. In this embodiment, the natural number K is preferably configured as 4. Of course, in other embodiments of the present invention, according to actual needs, K can also be configured as other reasonable natural numbers (such as reasonable values in [5, 10], etc.), and the present invention is not limited thereto.
[0067] In step S302, the network parameters of the initial neural network are set to random numbers, and the initial error data is set to infinity, and in step S303, the outer loop flag i is set to 0, and the inner loop flag k is set to 1.
[0068] In step S304, the average network parameter of the i-th round is used as the initial value of the neural...
PUM
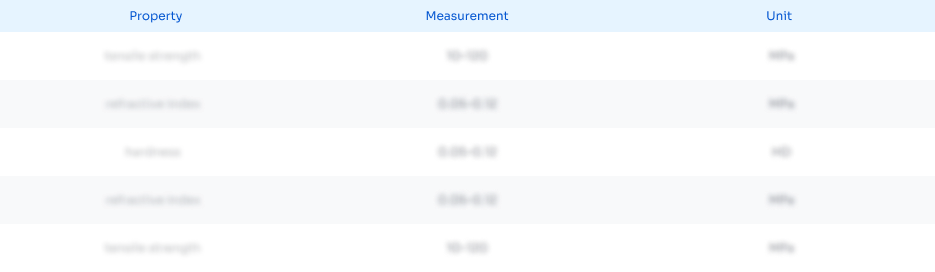
Abstract
Description
Claims
Application Information
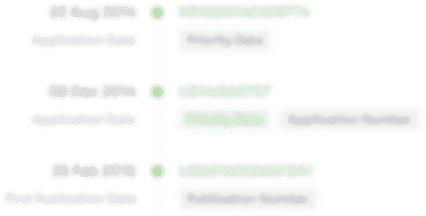
- Generate Ideas
- Intellectual Property
- Life Sciences
- Materials
- Tech Scout
- Unparalleled Data Quality
- Higher Quality Content
- 60% Fewer Hallucinations
Browse by: Latest US Patents, China's latest patents, Technical Efficacy Thesaurus, Application Domain, Technology Topic, Popular Technical Reports.
© 2025 PatSnap. All rights reserved.Legal|Privacy policy|Modern Slavery Act Transparency Statement|Sitemap|About US| Contact US: help@patsnap.com