Attention mechanism-based in-depth learning diabetic retinopathy classification method
A diabetic retina and deep learning technology, applied in the field of deep learning diabetic retinopathy classification, can solve the problems of poor robustness, no lesion distribution information into consideration, cumbersome manual feature extraction process, etc., to achieve good robustness and improve classification performance Effect
- Summary
- Abstract
- Description
- Claims
- Application Information
AI Technical Summary
Problems solved by technology
Method used
Image
Examples
Embodiment 1
[0030] Embodiment 1: The attention mechanism-based deep learning diabetic retinopathy grade classification method provided by the present invention detects and recognizes the grade of diabetic retinopathy, and the specific operation is carried out as follows:
[0031] 1. Select the data set;
[0032] (1) EyePACS dataset
[0033] The diabetic retinopathy five-category dataset contains 88,702 color fundus images from 44,315 patients, and the resolution of the images is between 1 and 2. The data set is divided into two parts: training set 35126 (from 17563 patients), test set 53576 (from 26788 patients). The DR severity level of each fundus image is marked by the doctor according to the ETDRS table: '0' means no diabetic retinopathy, '1' means mild non-proliferative diabetic retinopathy, '2' means moderate diabetic retinopathy, '3 'Indicates severe diabetic retinopathy,'4'indicates proliferative diabetic retinopathy. The EyePACS dataset has the following characteristics: (1) A...
PUM
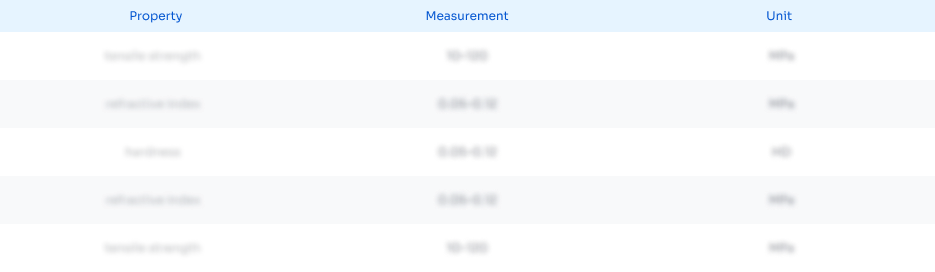
Abstract
Description
Claims
Application Information
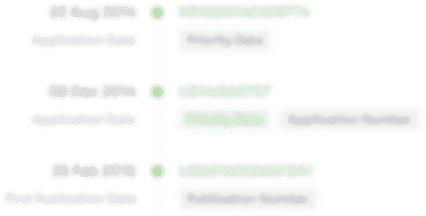
- R&D Engineer
- R&D Manager
- IP Professional
- Industry Leading Data Capabilities
- Powerful AI technology
- Patent DNA Extraction
Browse by: Latest US Patents, China's latest patents, Technical Efficacy Thesaurus, Application Domain, Technology Topic, Popular Technical Reports.
© 2024 PatSnap. All rights reserved.Legal|Privacy policy|Modern Slavery Act Transparency Statement|Sitemap|About US| Contact US: help@patsnap.com